There are four main data structures: structured data, unstructured data, semi-structured data, and metadata.
Structured data is information that is formatted and organized for readability within relational databases.
On the other hand, unstructured data’s format is undefined, not well-organized, and not usable by relational databases.
In this article, I will explore structured vs. unstructured data, semi-structured data, how to convert unstructured data, and AI’s impact on data management solutions.
What is structured data?
Structured data is highly organized data formatted to integrate with a relational database easily. Structured data resides in tabular formats, such as SQL databases.
As I mentioned previously, structured data is typically collected from spreadsheets and DBMS systems and is primarily, but not always, quantitative in nature. Because structured data complies with relational databases, it also easily integrates with AI technology, specifically machine learning.
While structured data is widely regarded as the most sought-after and user-friendly data type, structured data is the least common data structure type. This is because structured data is collected from spreadsheets, relational database management systems (RDBMS), etc.
If we were to think about where most of our data is collected, we would notice that public web sources and other more informal collection methods are the most common, even in the world of finance.
However, while structured data accounts for only about 20% of total global data, it is still extremely valuable, particularly to companies and investors. For example, secondary data usually falls under the 20% of cleaned and structured data.
Let’s take a closer look at structured data.
How is structured data managed?
The management of structured data was created by IBM in the 1970s. IBM needed to develop a language that defined how each data point within a larger database relates to one another, creating the Structured Query Language, known more widely as SQL.
This language helped companies replace outdated paper-based business intelligence processes with digital processes. This digitalization has since enhanced data analysis and improved business operations by reducing costs and improving efficiency, among other benefits.
Today we are seeing companies leverage the digitalization of such structured data for building AI-based tools and data-driven decision-making.
Structured data is well-organized and formatted, making it easy to find in relational databases. Unstructured data is difficult to capture, process, and evaluate since it lacks a predefined format or organization.
- Jason Mitchell, CTO of Smart Billions

What is unstructured data?
Unstructured data is not organized or formatted in a predefined data model. It is stored as media files or NoSQL databases.
Typically qualitative in nature, unstructured data includes a variety of data types such as text, numbers, booleans, and enumerations. Because unstructured data is collected from a variety of sources, raw unstructured data in its unfiltered form is disorganized and complex. Therefore, it's harder to analyze unstructured data.
Despite its complexity, unstructured data, when integrated, organized, and analyzed properly, provides companies with high-quality qualitative data and business insights. This then raises the question, if unstructured data is so complicated, why is it so sought after?
The answer is simple: unstructured data accounts for approximately 80% of data worldwide.
More specifically, unstructured data is sourced from a variety of sources, including social media, documents, PDFs, audio files, video files, sensor data, and more.
The variety of source types and loose formatting definitions require unstructured data to be processed and transformed for further analysis or integration.
How is unstructured data managed?
As I mentioned before, unstructured data accounts for the majority of data while also being the most complex, unorganized, and typically largest in size. However, because it is so valuable, data scientists have been looking for unstructured data management and storage solutions.
Today, AI technology, automation, and cloud technology have been the key to such solutions. More specifically, unstructured data is stored using servers and cloud-based technology and is managed with natural language processing and data mining.
Still, some companies refuse to manage unstructured data. They simply expand the storage space and fill it with unstructured data. However, unstructured data requires management in order to save storage space and have the ability to ingest other types of data.
What is semi-structured data?
Semi-structured data is a combination of structured and unstructured data.
Semi-structured data has some organization and formatting but not enough to integrate into relational databases smoothly. Semi-structured data’s organization is minimal and is organized with tags, attributes, and other semantic markers.
However, the resulting organization does not meet the standard of a relational database but can be altered in a way that can fit into easy-to-read tables and spreadsheets, making it a subset of unstructured data.
How is semi-structured data managed?
Similar to how unstructured data is managed and stored, semi-structured data is managed using servers, cloud-based technology, natural language processing, and text data mining.
Ultimately, as we create more and more unstructured and semi-structured data, we will begin to see new trends in data management solutions.
Structured vs. unstructured data: key differences
Let’s take a closer look at the key differences and similarities between structured, unstructured, and semi-structured data.
Converting unstructured data
As our global data sphere continues to grow, businesses will look for solutions to unlock the full potential of all data structures. Currently, data scientists can extract insights from unstructured and semi-structured data using a conversion process.
Let’s take a quick look at this process.
How to convert unstructured data
1. Analyze your data sources
Before you begin the conversion process, you must identify and analyze what data you want to convert. This will require that you access a data lake, where raw unstructured data is stored, and decide what datasets to pull from.
2. Set clear goals and objectives
This step can be done in tandem with the first step. Setting clear goals and objectives about what you want to extract from your data and how you might use and access it in the future will provide guidance for the rest of the process.
3. Evaluate and select the processing tools
Once there are clear objectives, and your data sources have been analyzed, you can select which processing tools will work best. These processing tools include text mining, data extraction tools, and natural language processing tools.
4. Clear and tag the data
Now that you have chosen the processing tool, you must clean and formulate the data according to the chosen tool’s guidelines. This might involve deleting extraneous symbols, whitespaces, and duplicate data.
This step will help your processing tools understand the basic organization and entities within your data.
5. Data extraction: Text mining & Natural language processing (NLP)
The final step involves running your data through the selected processing tool: text mining or NLP. Text mining involves sifting through textual data for significant words and phrases and extracting key features of each document or data file.
NLP involves utilizing AI to sift through and decipher the natural languages (human languages) within textual data.
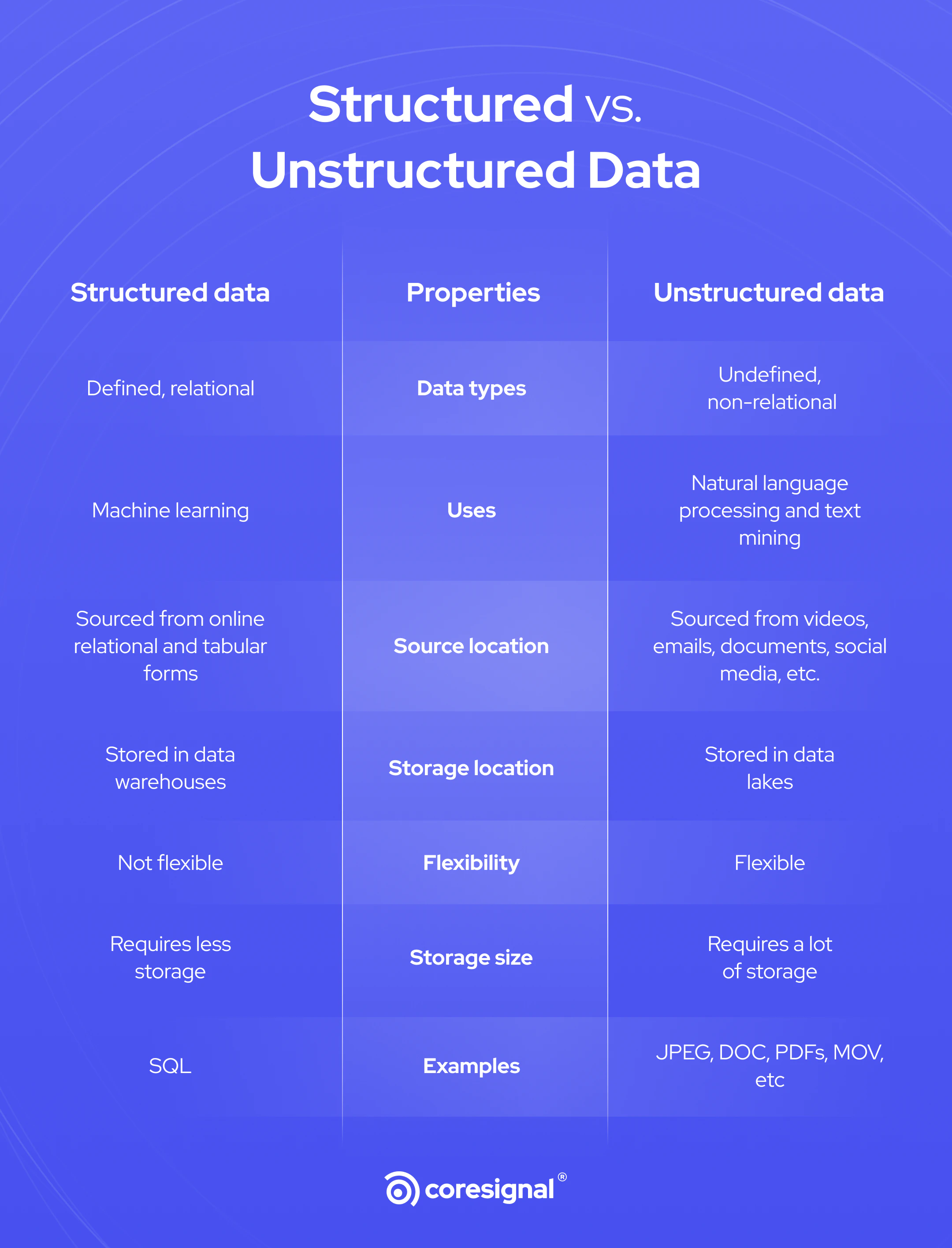
Data structures and AI
Our global data footprint is expanding at an exponential rate. According to a study by Seagate, the global data sphere is expected to reach 175 zettabytes by 2025, a massive growth from 45 zettabytes reported in 2019.
As our data footprint grows, companies are finding more ways to leverage this data for crucial business practices such as lead generation, market analysis, and business and investment intelligence, just to name a few.
Coresignal's data also helps with the aforementioned practices.
Data structures and AI
Our global data footprint is expanding at an exponential rate. According to a study by Seagate, the global data sphere is expected to reach 175 zettabytes by 2025, a massive growth from 45 zettabytes reported in 2019.
As our data footprint grows, companies are finding more ways to leverage this data for crucial business practices such as lead generation, market analysis, and business and investment intelligence, just to name a few.
Likewise, as our data grows, the challenges surrounding the usability and hidden potential of data have become apparent. This is primarily due to the varying structures of data that are created and consequently collected.
Ultimately, data scientists have turned to AI and machine learning for these solutions.
Further, artificial intelligence utilizes both structured and unstructured data. More specifically, structured data utilizes machine learning, while unstructured data utilizes text mining and natural language processing (NLP), all of which are AI-based processes.
Scientists have created AI-based tools that can extract valuable business insights from nearly all data structures.
For example, companies can purchase semi-structured data from data providers, prepare said data for analysis or enrich their current datasets, and then extract valuable insights from the data with AI, analysis, or other automated data processing tools.
Structured data is utilized in machine learning and drives machine learning algorithms. Unstructured data is used in text mining and natural language processing.
Veronica Miller, Cybersecurity Expert at VPN overview
Top tools for structured and unstructured data analysis
Structured data tools
Schema. Best for content marketing teams that have little to no technical knowledge.
Google's Structured Data Testing Tool. It tests whether your URL or a code snippet has any issues. It's very useful while installing schema into your website.
Merkle. It helps create data for articles, FAQs, breadcrumbs, and more. It also offers features such as SEO, SERP, and crawling, among others.
The RankRanger Structured Data Tool. It also assists in creating data for articles, FAQs, and more.
Unstructured data tools
Excel. An easy-to-use tool that allows for basic quantitative data analysis and visualization.
Tableu. A data visualization tool that allows making comprehensive data visuals for reporting.
RapidMinder. An advanced data science platform that is capable of building predictive models and is compatible with large amounts of data.
KNIME. An open-source data platform best for experienced IT professionals that are interested in creating specialized tools.
Power BI. An advanced data visualization tool for business intelligence.

Wrapping up
In all, understanding the various data structures is beneficial in informing companies about the importance of data management. In order to unlock the full potential of data, companies must maximize their data management and storage processes of both structured and unstructured data.
As our global data sphere continues to grow at exponential rates, data scientists and companies will continue to develop management and storage for all types of data structures.