Venture capital (VC) investments are often associated with psychology and a thorough decision-making process that can be affected by human error, as research suggests. Traditionally, investors use intuition and deduction to analyze and pick startups, which is why more and more investment managers have recently started to seek external help in the form of public web data and artificial intelligence (AI). This article explores the benefits of public web data, AI and machine learning (ML) in venture capital and how it can help investors correctly identify rewarding opportunities.
The emergence of data-driven investments
While traditional investment models have been prevalent and successful, their caveats can turn disastrous. Theories have different shortcomings, such as false assumptions, that do not characterize real-world markets. As a result, the reality is more complex and has anomalies that are not considered in theoretical models.
Seeking more sophistication, investors have turned their attention to data-driven investments. Also referred to as public web data, this information comes from a wide range of non-traditional sources, often web-based. According to Greenwich Associates 2018 Alternative Data Customer Journey Study, 48% of investment managers access web-scraped data, while 45% choose other sentiment sources, followed by credit card and POS systems, search trends, and many others.
Public web data is listed as one of the five venture capital trends of this year. It is an important source of information because it is more varied than traditional data sources; for example, public web data can be used to identify numerous insights into any company regardless of its growth stage.
For instance, the American retail company JCPenney outperformed nearly all expectations in the second quarter of 2015, followed by an impressive rise in its share prices. However, a big data company known as RSMetrics obtained satellite imagery of its parking lots, showing that its stores’ traffic increased. Investors who bought this type of external data could see in advance that the company’s performance was growing more than the predicted values and could capitalize on this information.
How is machine learning used in investment decisions?
Machine learning is a branch of artificial intelligence. It refers to algorithms or models which are created to increase their accuracy over time by learning from data without the need for any further programming.
Investors now have access to more and more data thanks to technological advancements. Machine learning methods are used in order to sort or analyze large amounts of data while becoming more efficient and accurate over time.
When it comes to identifying investment opportunities, machine learning can help by collecting and analyzing public web data following models that can predict future rewards. A venture capital firm can use public web data and machine learning to assess the future success of startups.
Machine learning in high-frequency trading
Machine learning is also inevitably a part of high-frequency trading (HFT). HFT refers to algorithmic trading in which an impressive amount of orders are conducted in a short time, providing liquidity on the market. Given this simplistic definition of HFT, artificial intelligence seems to be the most efficient way to process all the information. These models show pricing increases or decreases in the market and then make the correct bids. Most HFT companies nowadays integrate machine learning into their processes.
Machine learning and signal generation
Another aspect developed in the past years using ML-based algorithms is signal generation. The traditional seasonal signals use historical price patterns to make predictions. When this process is powered by artificial intelligence, investors can generate better signals that include a wide range of additional data, such as volatility, sentiment, price action, structural changes, valuation metrics, and many more.
Given the abundance of such information, ML-based models can compute new trading signals. In addition to this, some models can make real-time predictions, and it continuously learns new data and adapts to the real-world market environment.
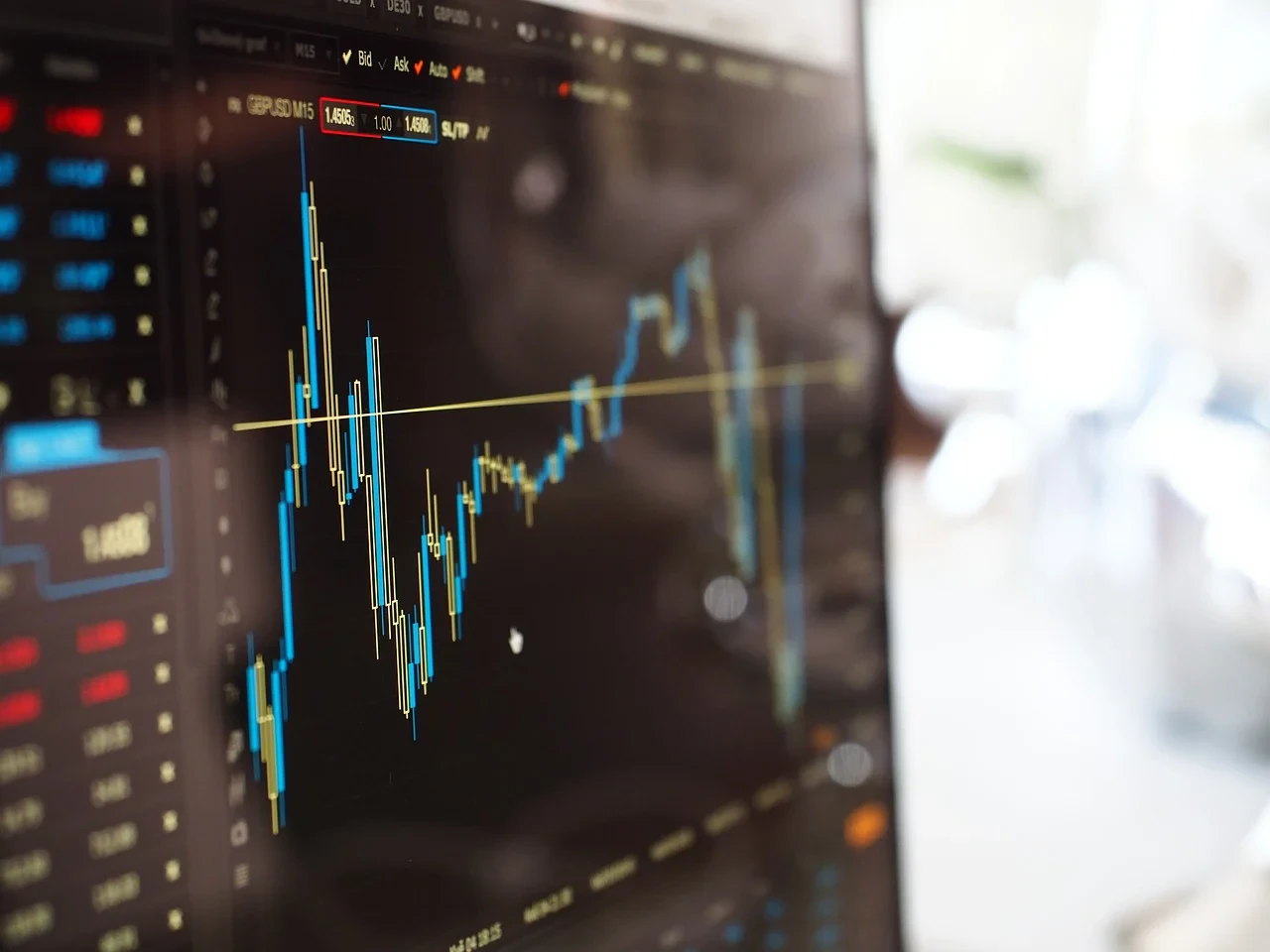
Why is machine learning important in venture capital?
Smart algorithms have become more and more important nowadays. From tech startups such as Kensho and Alphasense to Silicon Valley established companies, more and more investment firms implement data-related developments in their processes.
Unmatched efficiency
Hone Capital is a Silicon Valley-based company that partnered with Wellfound, a firm with great access to different data types. They created a machine-learning algorithm using more than 30,000 deals from the past ten years from different sources, including PitchBook and MatterMark.
This allowed them to analyze more than 400 characteristics, including the founders’ background, historical conversion rates, money raised, and more. This resulted in a list of top 20 companies with the most potential for future success. Such data-related developments in investments can help venture capital firms further curate long lists of startups and minimize or completely avoid losses.
In short, machine learning can help to identify rewarding opportunities with unmatched efficiency. Although venture capital companies subscribe to different databases that list numerous startups’ data, it is obvious that the human brain cannot process such impressive amounts of information.
Big data and human limitations
Cukier and Mayer-Schönberger published a book on big data called “Big Data: A Revolution That Will Transform How We Live, Work, and Think.” They discuss that humans cannot consider and compare three characteristics of three different products simultaneously. Despite this, a venture capital manager should consider factors regarding both past and future startups and the market, resulting in the need to make investment decisions that could be worth millions of dollars.
This is where AI and machine learning can improve the current practices and outcomes of VC investments. Since technology can quickly identify the optimal matches using data, venture capitalists can focus on suitable investments while eliminating startups with low or no return on investment.
Machine learning and competitive advantage
Some industry experts go as far as to suggest that implementing machine learning in venture capital can result in a significant competitive edge. This is because technology enhances the decision-making process that could differentiate between a bad investment and $15 million returns. This is consistent with research showing that machine learning methods help investors find better opportunities and reduce the uncertainty associated with venture capital.
To achieve long-term success, a venture capital firm must have a strong competitive edge. Researchers firmly state that strategic advantages are tightly related to information due to the current knowledge-based economy. In other words, better access and processing of information can lead to making better investment decisions and choices. As a result, artificial intelligence can make all operations quicker and more efficient while reducing human bias.
People's behavior can be predicted to a degree when you have many past examples, but there is a limit — what data would have predicted the success of Google in 1998 or Facebook in 2003?
- Gregory Piatetsky-Shapiro, KDnuggets
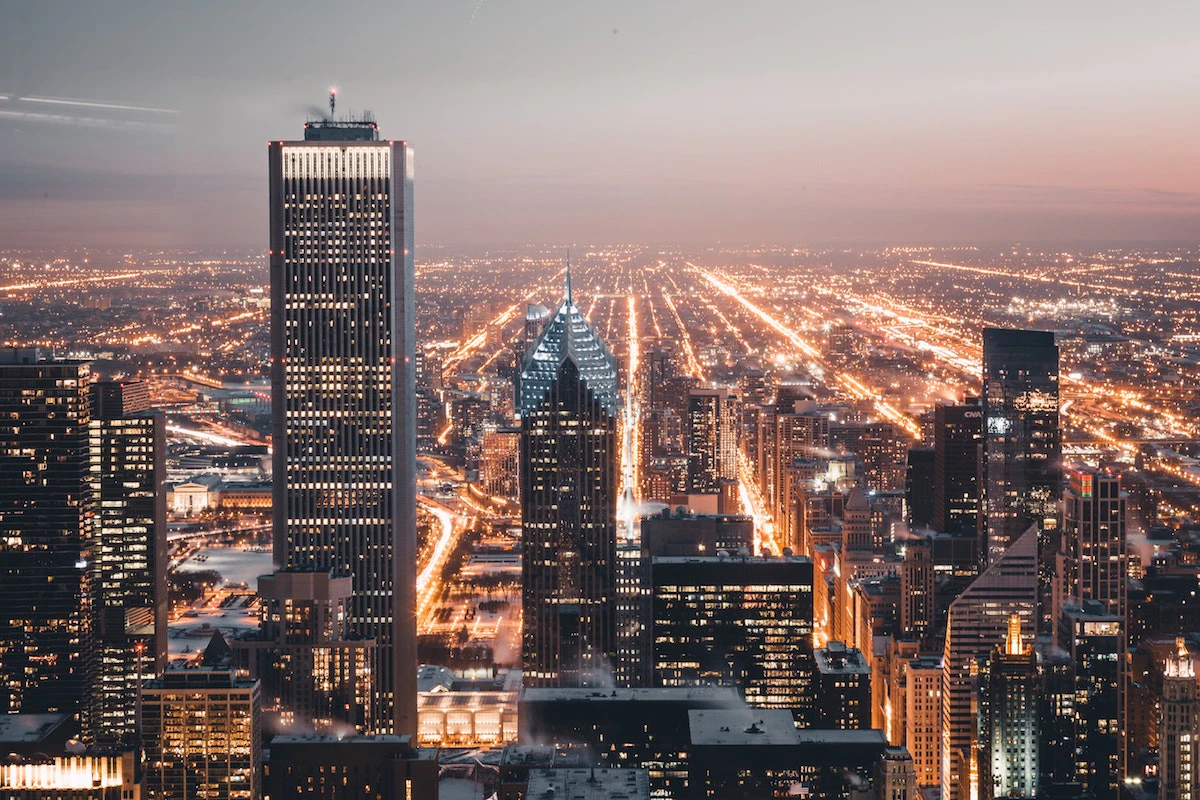
Five benefits and applications of machine learning in venture capital
1. Finding companies looking for funding
As briefly discussed above, one of the most obvious uses of machine learning is to screen startups ready for investment. This type of technology allows venture capitalists to filter a myriad of companies in different industries, verify whether they are seeking funding, if they already received investments, and many more. The list can then be curated using financial or other company-specific factors.
2. Identifying opportunities
Machine learning can identify new companies showing early signs of growth. These can be analyzed using indicators such as hiring plans and the expansion of their teams. Finding early-stage companies is crucial for VCs; machine learning helps to identify firms or products that are relatively new and receive impressive amounts of attention. This can be combined with social media data to conduct sentiment analysis.
Another alternative data application is to identify and track down elite professionals, graduates, professionals and their career paths. This information can indicate when a possible high-growth company is in its early stages. For example, machine learning can generate signals that inform VCs that several Ivy League graduates have just founded a company.
3. Timing and investment
One of the success factors in any type of investment is timing. Machine learning can help VCs identify companies’ growth stages so they can invest at the right time. For instance, technology can determine which teams are growing the fastest, the speed of growth, hiring strategy, business actions, and many more indicators.
4. Growth tracking
One of the relatively new investment strategies used in venture capital industry is growth tracking. Using machine learning, VCs can monitor how their existing portfolio companies are doing. Moreover, investors can analyze the company’s online presence to determine its popularity; this is conducted by monitoring online reviews, social media platforms, and even new job ads. Machine learning identifies companies that do well online, indicating future growth.
5. Satisfaction tracking
Finally, machine learning can monitor employee satisfaction. This can be a powerful indicator for VCs because employee dissatisfaction expressed in company employee reviews may indicate severe company or management issues. On the other hand, general satisfaction suggests that the company does not have any apparent problems.
Summary
All in all, machine learning is quickly gaining popularity in the investment world. Venture capital firms use artificial intelligence to adapt to the new knowledge-based society and reduce information asymmetry. AI and machine learning methods can ultimately help companies make data-driven venture capital investments and separate companies with low or no chances for success from those with impressive potential to generate returns.