Data-driven decision-making is perhaps one of the most talked-about financial and business solutions today. The success of such data-driven solutions requires a variety of data types.
On the one hand, there is traditional data, or internal data, produced by a particular company. On the other hand, there is non-traditional, or web data, collected from numerous external sources.
In this article, I will focus on web data and provide a deeper understanding of the nuances of web data types.
What is the difference between qualitative and quantitative data?
- Qualitative data. Non-numerical data that provides information for qualitative research methods. It's usually unstructured textual information that allows gathering information about the quality of a product or service. For example, it could be product reviews, company employee reviews, surveys, and more. Qualitative research aims to answer the question why.
Quantitative data. Numerical data that provides information for quantitative research methods. It could be structured more easily and put into graphs and charts for better readability. This type of data shows numerical values such as company revenue, headcount, funding amount, and more. Quantitative research aims to answer the question what.
Types of Data
Types of data in statistics and analysis can vary widely – and, as a result, create confusion. Some of them, like quantitative and qualitative data, are different concepts.
Other types of data include numerical, discrete, categorical, ordinal, nominal, ratio, and continuous, among others. However, all data types fall under one of two categories: qualitative and quantitative.
Let's take a look at these two data types.
Qualitative Data
Qualitative data refers to interpreting non-numerical data. It is often unstructured or semi-structured, and perhaps one of the easiest ways to identify it is that it does not come as numbers.
In other words, the qualitative approach refers to information that describes certain properties, labels, and attributes.
Qualitative methods are often known as investigative as they can be used to answer the question why using open-ended questions. It helps create a story, develop hypotheses, or obtain an initial understanding of a case or situation.
Sources of Qualitative Data
Qualitative data is generated via numerous channels, such as company employee reviews, in-depth interviews, and focus groups, to name a few.
This type of web data often comes in an unstructured form and is often difficult to collect and analyze.
Some examples of qualitative web data include information collected from social media, search engines, product reviews, comments, or other web interactions.
Interested parties can collect these data directly from the source (i.e., social media platforms), or utilize web data providers.
The benefit of choosing a data provider is that the information is already selected and presented in an easy-to-understand format, rather than collecting all the data available on all social media platforms or search engines.
For example, with company employee review data, you can see the internal environment of a company and identify potential risks. The reviews are written by employees who work in the company, therefore it helps you assess the internal situation. If the reviews are negative, it might indicate problems in the company and make you think twice about investing in it.
On the other hand, if the reviews are positive and the employees are happy to work there, it indicates that the company takes care of its employees. As a result, it might solidify a potential investment opportunity.
Examples of qualitative data
Examples of qualitative data that might interest investors and businesses are extremely varied. These depend on your objectives, the scope of the research project, and the purpose of your data collection.
For instance, if you want to invest in a business, you may be interested in the comments on social media that mention the company's products and whether the review is positive or negative.
Alternatively, a company trying to gain an insight into their competitors might seek the same information or may want to find out the socioeconomic status of their clients.
Another source of qualitative data when it comes to web data is sensors. This refers to information collected from CCTV, POS, satellites, geo-location, and others.
This data collection is facilitated via the interconnectivity of devices. It could indicate, for instance, the foot traffic at the competitor's business location.

Main types of qualitative data
Categorical data
As briefly mentioned above, some data types refer to the same information. In statistics, qualitative data is the same as categorical data. This is because this information can be easily categorized based on properties or certain characteristics.
The main feature is that qualitative data does not come as numbers with mathematical meaning, but rather as words. In some cases, qualitative data may be assigned numbers (1 or 0, for instance) for analysis purposes.
For instance, if you conduct a questionnaire to find out the native language of your customers, you may note “1” for English and “0” for others.
However, these numbers have no meaning from a mathematical perspective; similarly, if you check the postcodes of your clients, the data is still qualitative because the postcode number does not have any mathematical meaning; it only shows the address of your customers.
Nominal vs. ordinal data
Categorical data can be further split into nominal or ordinal data.
Nominal data refers to information that cannot be sorted in a given way – you can assign categories to these data, but you cannot order them, for instance, from the highest to the lowest.
For example, information collected through “yes” or “no” closed questions is a type of nominal data: “would you recommend this product?”. The answers collected can be split into “yes” or “no”, but you cannot further organize them.
Nominal data helps you calculate percentages, such as 50% of comments on social media were happy with the company's after-sale service, proportions, or frequencies.
The opposite type of categorical data is ordinal; in other words, you assign categories to your qualitative data, and then you can order them in a logical way.
Let's assume that you have a B2B company and you want to collect information about your clients. You can obtain firmographic data indicating the size of each client company and assign them to small, medium, or large enterprises. This is a type of ordinal data. Unlike the information with “yes/no” answers, the categories can be ordered from small to large.
Ordinal data can also be assigned numbers; however, these have no mathematical meaning. Thus it is still under the qualitative umbrella. For example, if you conduct a questionnaire asking customers to rate the quality of a product from 1 to 5, with one being “poor” and five being “high-quality,” your ordinal data can be categorized and assigned to these numbers.
However, from a mathematical perspective, they do not have any meaning. It's rather just a simple way of sorting the data. Just like nominal data, this can also be used to calculate percentages, proportions, and frequencies, among others.
Benefits of qualitative data
Qualitative data helps you understand the reasons behind certain phenomena. For example, you notice that your competitor's revenues are 50% higher than yours. The quantitative data, such as revenue numbers, does not help you understand why the company performs much better.
The right qualitative data can help you understand your competitors, helping you adjust your own competitive strategy to stay ahead of your competition.
For example, you can use data collected from sensors to identify the foot traffic at your competitor's location. In this case, you may find out that they have more customers than you do, which explains the revenues.
Alternatively, you may find the same amount or fewer customers, which may mean that they charge a premium for their products and services.
Some other benefits and applications of such web data include:
- Fine-tuning marketing strategy by collecting ideas or opinions from social media platforms;
- Look for ways to attract new customers;
- Obtain a granular insight into a business or your chosen target audience;
- Stay on top of the competition by becoming familiar with other companies' business strategies, strengths, and weaknesses;
- Obtain detail-oriented data to inform investment or business decisions.

Quantitative data
The second major type of data is quantitative. As the name suggests, it is data in numbers with mathematical meaning that indicate quantities of specific aspects. For example, a company's financial reports contain quantitative data. The main benefit of quantitative data is that it is easier to collect, analyze, and understand than qualitative data.
One can easily visually represent quantitative data with various charts and graphs, including scatter plots, lines, bar graphs, and others. You can also collect quantitative data to calculate ratios, for instance, if you want to compare a company's performance or study its financial reports to make an investment decision.
Sources and examples of quantitative data
Web data of this type can also come from a variety of sources. You may use market reports, conduct surveys, or collect web scraped data that can be transposed into numbers with certain values. Some examples include the number of web visitors, a company's total number of employees, and others.
Some examples of quantitative data include credit card transactions, sales data or data from financial reports, macroeconomic indicators, the number of employees or the number of job postings, and many more.
Types of quantitative data
Discrete vs. continuous data
Discrete data refers to certain types of information that cannot be divided into parts. For example, a company cannot have 15.5 employees – it's either 15 or 16 employees. In simple words, discrete data can take only certain values and cannot include fractions.
On the other side, continuous data can be divided into fractions and may take nearly any numeric value. For instance, a company's net profit of $100593,74 is continuous data. The same happens with the financial information of a company, such as sales data, credit card transactions, and others.
Benefits of quantitative data
Quantitative data is easy to interpret and can be collected easier because of its form. Some of the main benefits of collecting quantitative data depend on the type of information you seek. For instance, firmographics, or firm-specific data, allows you to have a quick glance at your competitors' size, employee numbers, and others.
Although quantitative data is easier to collect and interpret, many professionals appreciate qualitative data more. However, this is primarily due to the scope and details of that data that can help you tell the whole story. Likewise, quantitative data is oftentimes favored due to the ease of processing, collection, and integration.
Some of the main benefits of quantitative data include:
- Easier and quicker to collect;
- More objective and accurate since it's expressed in numbers;
- Easier to categorize, organize, and analyze;
- You can obtain larger data samples;
- Suitable for statistical analysis and AI-based processes;
- Obtain detail-oriented data to inform investment or business decisions.
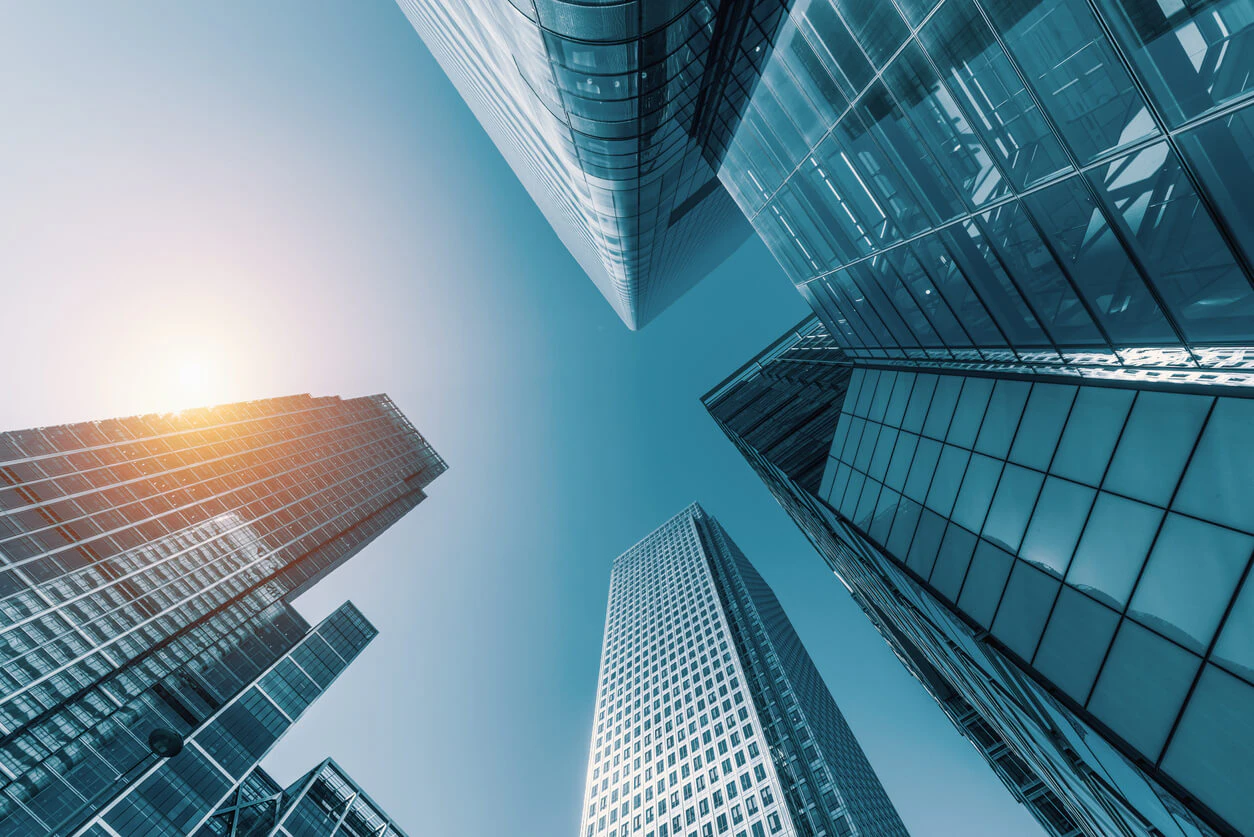
In short
Similarities between qualitative and quantitative research
- Both research types require raw data;
- Both are used to measure results;
- Sometimes one type of research complements the other.
Differences between qualitative and quantitative research
- Qualitative researchers seek non-numerical information, quantitative researchers analyze numerical data.
- Qualitative research is harder to draw results from because of unstructured data, whereas quantitative data is more structured.
- Qualitative research is based more on subjective views, whereas quantitative research shows objective numbers.
- Quantitative data allows for both inferential statistics and descriptive statistics, whereas with qualitative data you can only do descriptive to a limited extent.
When to use each?
If the situation allows it, it's best to use both to see the full picture. However, they can be also successfully used individually.
Qualitative research is best when the goal is to collect data about a product's or service's satisfaction between users. It can help improve your product intelligence and find weak spots that can be improved.
Quantitative research is best when the goal is to find new companies to invest in, for example. It's scaleable and automation-friendly. Selecting a numerical value of headcount would help you find a list of ideal companies that fit your investment criteria. There are many other factors that contribute to it, from funding rounds and amounts to the number of social media followers.
Conclusion
Both types of data help answer different research questions. Qualitative questions focus more on social research design and textual answers from control groups so businesses can personalize content and products to better fit the target audience, among other things.
Quantitative questions focus more on data in the numerical form to identify patterns and describe findings in charts, among other things.