The widespread application of quantitative investment strategies is a relatively recent trend. Over the past few decades, the field of quant investing has made significant advancements in the world of finance. Additionally, the field has been evolving to create new investment technologies that ultimately simplify the process. This article will provide you with a better understanding of quant investing and how it can be implemented for better decision-making and increased portfolio returns. Let's start by taking a closer look at the evolution of quantitative investing.
The emergence of quantitative investing
Sam Eisenstadt established the roots of quant investing in 1965. He created the first quantitative ranking system using 6-month trailing performance and discovered that the top stocks were outperforming the bottom-ranked stocks.
Nowadays, most of the investment community has adopted quant investment strategies. Many funds and institutional investors use them to outperform stocks and increase their returns. Let’s have a closer look at quantitative investing and why it attracted the attention of many investors.
What is quantitative investing?
Quantitative investing, often called systematic investing, refers to adopting investment strategies that analyze historical quantitative data. You can conduct data analysis and use advanced models to calculate probabilities and identify the optimal moment to make profitable investment transactions.
Quant investing consists of two essential parts: research, which could be based on proprietary research, and implementation.
What is a quant investing strategy?
A quant investing strategy is an advanced mathematical model developed by industry professionals, including programmers, statisticians, and investment analysts. The purpose is to identify stocks with a higher probability of outperforming an index using a broad range of characteristics. Different models are available and may consider various factors, as we discuss in the next section below regarding different types of investing strategies.
On a side note, quantitative techniques also help with asset allocation and risk management as well as aligning portfolios according to the needs of the clients.
Early adopters are now investing in public web data sources and methods to interpret large amounts of information using machine-learning models. This data, as opposed to traditional data sources, comes from web scraping which refers to collecting data from websites. Machine-learning models are a branch of artificial intelligence (AI) that allows you to compile and interpret a large volume of information to make better investment decisions.
Narrowing down the scope of public web data, historical headcount data could be one of the metrics to track. With this information, you can draw up a graph and see the changes in headcount in a company to determine its growth. Headcount data allows you to see how well a company is doing in terms of its size and hiring tendencies. A stagnant graph line might indicate that the company is standing still and not making any significant moves or expansion decisions.
For instance, you can see Tesla's headcount data below and decide for yourself what insights you can gather. No words are better than getting to see the data used in practice.
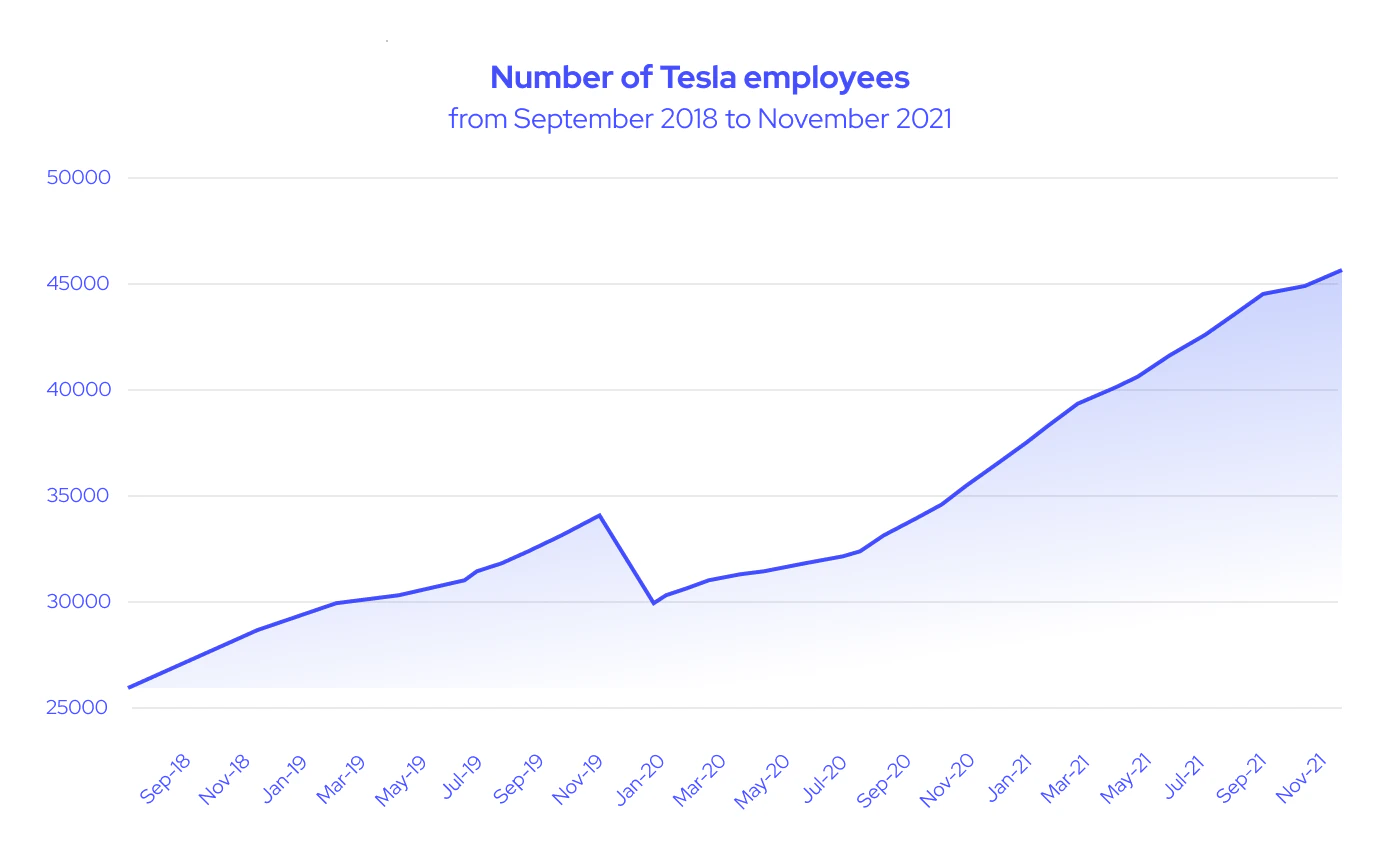
Quantitative investing, often called systematic investing, refers to adopting investment strategies that analyze historical quantitative data.
Types of quantitative investment strategies
Most quantitative strategies are known as relative value or directional. One aspect these strategies have in common is that they use software and computer models to predict outcomes using historical data. Quantitative investing is also known as data-driven investing.
Relative value quant strategies aim to identify pricing relationships and capitalize on them. For example, investors may use a model that finds a predictable pricing relationship between short-term government bonds and long-term government bonds.
Directional strategies follow trends or other patterns that might suggest price increases or decreases. For instance, these aim to find historical evidence using quantitative data to increase the long-term government bond prices in the future.
Some common quant investment strategies:
- Quantitative value strategy uses all the information in a company’s income statement and balance sheet. The model calculates an aggregated score and ranks equities;
- Event-driven arbitrage refers to strategies that analyze data regarding events, such as changes in regulations, corporate actions, and more. Buying and selling transactions occur if the model establishes a specific pattern in price movements;
- Risk parity funds refer to the concept that gains in one asset class offset losses in another asset class. This strategy may improve risk-adjusted returns over a long period;
- Passive investors use smart beta strategies (i.e., in mutual funds or ETFs) to improve the risk-adjusted returns using other factors than the market cap;
- Statistical arbitrage seeks to identify misplaced securities using the relationship between them. This strategy often employs financial ratios to open short and long positions. It's one of the active trading strategies;
- Investors initially used managed futures strategies on future markets to follow the major trends on the market. Nowadays, they have become more common in the stock markets, too;
- Factor-investing strategies use one or more factors that led to outperforming a benchmark index in the past. Some examples include growth, momentum, market cap, and value. The mathematical model scores each stock according to these factors and then uses the aggregate score to rank each of them;
- Systematic global macro strategies seek to identify countries and regions with favorable fundamentals. In other words, the model allocates the funds by analyzing the economy in various areas around the world;
- AI and big data strategies are the newest types of quant strategies. Generally, AI investing involves utilizing public web data. Additionally, it is important to note that research has reported that machine-learning-based quant strategies tend to be more efficient than traditional quantitative investments.
- Multi-asset strategies refer to combining several different types of assets into one diverse portfolio. The types of assets could range from stocks and bonds to real estate or cash.
Most quantitative strategies are known as relative value or directional.
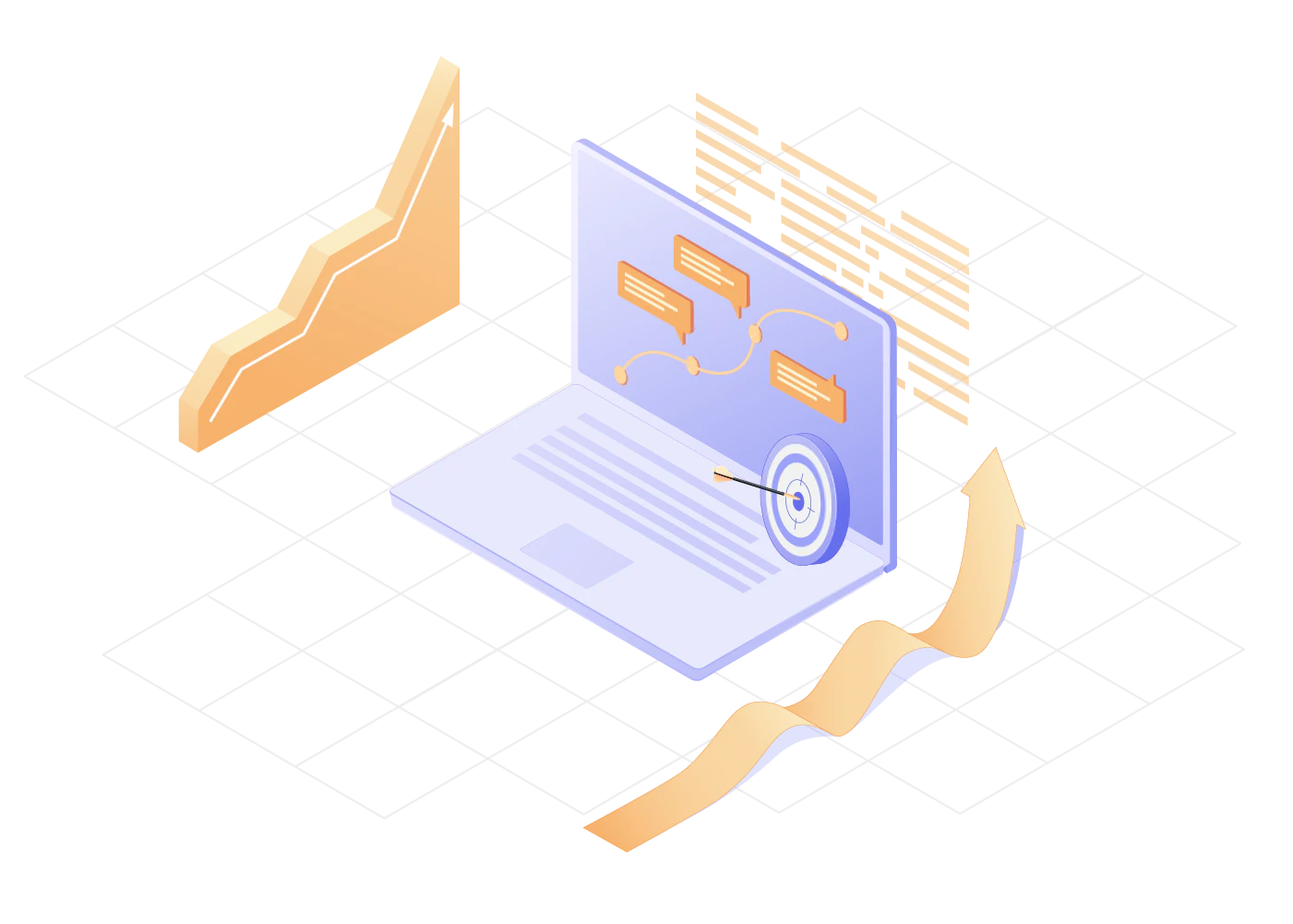
Benefits of quant investing
Now that we have unpacked the more common investment strategies, let’s see why investors are increasingly adopting these strategies.
Consistent and reliable
Quantitative trading does not include emotional or psychological factors. Since a computer ranks and makes investment decisions, historical data and numbers are the only considered factors, so they are highly consistent. It makes quantitative models more reliable and allows for better risk management since there is no room for human error in terms of calculations.
Cost-efficient investing
Given that there is no human input apart from developing the model, quant investing is more cost-efficient than other investment types. There is no need to hire experienced analysts or portfolio managers. Computers analyze all the data available and then make the transactions.
Easier to match the investor’s profile
Since quantitative analysis uses only historical numbers, investors can more easily predict risk and expected returns. This makes it easier to match a particular risk profile or create a portfolio for specific needs.
A larger pool of securities
Because a complex mathematical model conducts the process, there is no need for a sizable quantitative analyst team to identify outperforming stocks. Furthermore, investors can adjust the model according to relevant variables and apply it to any market and any volume of securities.
Overall
Given these benefits, it is clear that quantitative investors increasingly consider the clear potential of public web data and machine-learning methods to generate improved returns. Nowadays, most if not all strategies use software and advanced mathematical models to rank financial assets and make investment decisions on your behalf. To understand how this works, let’s examine quant investing strategies.
Quant investing offers the following benefits: consistency, reliability, cost-efficiency, easier predictions, and identification of outperforming stocks.
Challenges of quant investing
Shortcomings of using historical data
Like any other model or theory developed by humans, the quantitative investment strategy used is as efficient as the person who created it. One famous example is Long-Term Capital Management, a quant hedge fund that was extremely successful during the 1990s.
Led by Nobel prize winners Robert C. Merton and Myron S. Scholes, the fund attracted funds from all types of investors and enjoyed extra returns by identifying and exploiting market inefficiencies. Unfortunately, the model they used did not consider the possibility that the Russian government may default on its debt. When this happened, its founders had to liquidate it.
Since the quant hedge fund had deep roots in the world markets, its collapse had dramatic consequences. The Federal Reserve, other investment funds, and even banks had to intervene to support the fund from causing more damage.
Quantitative funds
Quantitative strategies require a relatively high amount of holdings since it's based on expected returns and probabilities. Also, they demand extensive periods of time to perform, and under the circumstances of a shorter time period, they will most likely underperform. However, this does not apply to all quant funds and additional data sources are employed to enable the short-term generation of alpha.
Sudden changes
There are unexpected changes or situations that only humans might recognize – such as a corporate scandal or management change that computers cannot identify. Although, one workaround could be implementing a mention tool in your system that could track negative sentiment and provide you with data to identify some of the changes.
However, once qualitative data is finally enabled in AI models, these issues will no longer be a problem.
The future of quantitative investing
Nowadays, numerous investors focus on technology and, particularly, machine-learning methods; for instance, more research focuses on the benefits of machine learning methods in venture capital. When used together with the investment strategies mentioned earlier, analysts may uncover relationships and patterns that haven’t been used before.
This has the potential of leading to significant improvements in the predictive models generated by quant investing models while minimizing their shortcomings, such as enabling artificial intelligence to include qualitative data in its models.

Summary
Quantitative investing is a systematic method that uses evidence-based data to make investment decisions. This lowers the overall costs of investments, requires less labor, and ultimately minimizes human input in the process. While a quant strategy is not a fool-proof one, the advances in artificial intelligence and big data indicate that the industry’s future may soon uncover unexpected opportunities for investors.