Professional network data
Leverage our top B2B datasets
Job posting data
Get access to hundreds of millions of jobs
Employee review data
Get data for employee sentiment analysis
Clean dataNEW
Enhanced professional network data
Employee data
Get data on global talent at scale
Funding data
Discover and analyze funding deals
Firmographic data
Unlock a 360° view of millions of companies
Technographic data
Analyze companies’ tech stacks
BY INDUSTRY
MOST POPULAR USE CASES
Investment
Leveraging web data for informed investing
HR tech
Building or enhancing data-driven HR tech
Sales
Supercharging your lead generation engine
Marketing
Transforming marketing with web data
Market research
Conducting comprehensive market research
Lead enrichment
Use Coresignal’s data for enrichment
Talent analytics
Analyze talent from multiple perspectives
Talent sourcing
Comprehensive talent data for recruitment
Investment analysis
Source deals, evaluate risk and much more
Target market analysis
Build a complete view of the market
Competitive analysis
Identify and analyze competitors
B2B Intent data
Lesser-known ways to find intent signals
BY INDUSTRY
MOST POPULAR USE CASES
Investment
Leveraging web data for informed investing
HR tech
Building or enhancing data-driven HR tech
Sales
Supercharging your lead generation engine
Marketing
Transforming marketing with web data
Market research
Conducting comprehensive market research
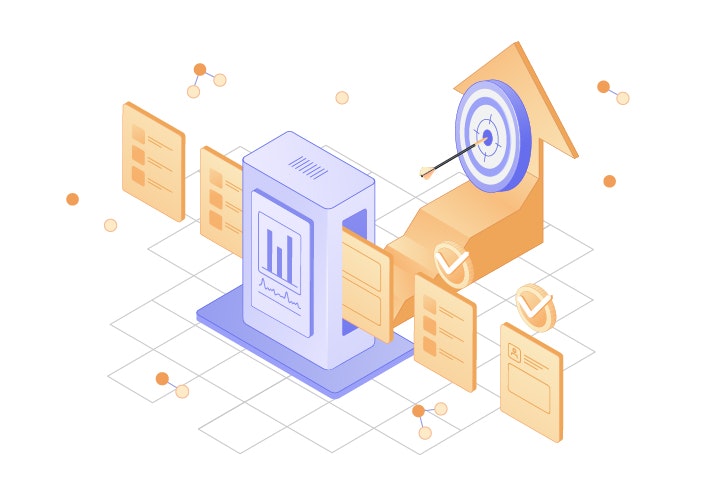
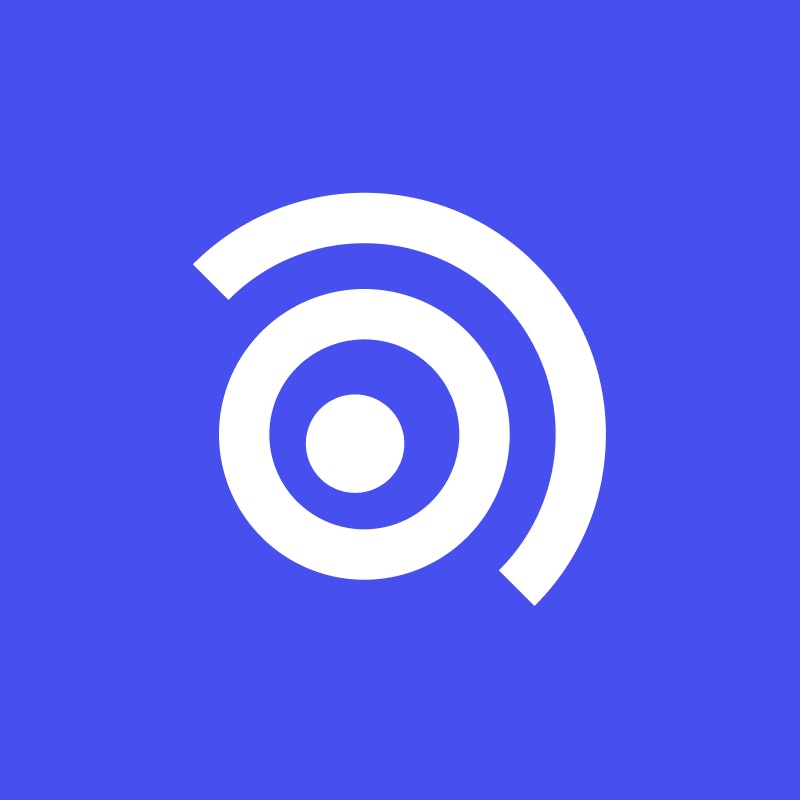
Coresignal
March 28, 2024
Raw data refinement is a vital step everyone should take before delving into the analysis stage. Skipping it means leaving your data team knee-deep in a pool of uncategorized, disparate, and often irrelevant information. Of course, you shouldn’t overdo this to avoid throwing away the valuable pieces.
However, not every business has the necessary resources to refine data on its own. In such a case, getting an already refined dataset could be the best option, bringing high value and low maintenance.
What is refined data?
Just as the name implies, refined data, also known as clean or filtered data due to the lack of unanimous terminology among the data science community, is the processed version of the raw data. It no longer has outliers, stylistic code tags, low-value records, or other unwanted elements. The refining process also involves removing duplicates and standardizing all values.
Broadly speaking, refined data is the opposite of raw data, from which it is “made.” To better illustrate the difference, let’s compare these two side-by-side.
Raw data vs Refined data
Feature | Raw data | Refined data |
---|---|---|
Filtering | All records | Complete, deduplicated records |
Standardization | No | Yes |
Text field cleaning | No | No code tags, special or trailing characters, double spaces |
Data points | Unaltered | Fewer data points due to filtering |
As you can see from the table above, the amount of data refinement shapes the new, cleaner, and leaner database. From the business perspective, the more data you want to save while processing, the more resources it will take to handle it. On the other hand, chopping instead of slicing may bring misleading results.
Data refinement process
Data refinement is a long and tedious process involving multiple steps, some of which might need to be repeated before reaping the benefits. Its core goal is to transform raw data into understandable and relevant information that data analysts can work with.
The number of steps and their names vary within the industry, but the ones below are found virtually in every source and are required to complete the data refinement process.
1. Removing irrelevant and duplicate data
The first step requires you to decide what data you actually need. Let’s say your product is oriented to large enterprises, so you start by omitting all businesses with fewer than 250 employees. This makes all the future steps, including analysis, much easier and quicker.
Then, you may want to remove HTML tags, special characters, double-spaces, duplicates, and incomplete records. However, this is also the step where you might lose vital information, so double-check if you don’t want to start all over again after getting no results in the analysis stage.
2. Fixing data structure
This step is for the computers, which need standardized field entries to make proper calculations. While it’s obvious to a human that November 12, 1934, and 12/11/1934 mean the same date, the algorithm will find these as two separate types. Until the AI fully takes over the data refinement process, this step will probably remain the most tedious.
The same goes for other data points, such as time, address, phone number, or URL. Even if you have the formats unified, you need to check for typos, wrong capitalization, and similar forms of pain in the lower back.
Before moving on to Step 3, we must warn you again. In this stage, you may find odd, out-of-range numbers that don’t fit the rest of the records. While most likely it will be irrelevant, checking before removing is the rule.
3. Managing missing data
Inevitably, you will run into some empty data fields. Depending on your objective, you may need to remove the record. This can work if you have millions of data pieces, and losing some won’t affect the outcome.
If the absent data is vital for the analysis, you may want to enter an average number in that empty field. For example, if the employee profile is missing salary, you can take the 2023 Q3 average wage in the US—$59,384.
Finally, if you need that cell to remain empty, you should look for an algorithm that could work with missing values. Do this if your gut feeling says that throwing out the record would be a bad idea.
4. Confirming data correctness
For the last step, check if everything is in order and all other steps are fully completed. Now, see if there’s enough data to do a proper analysis. Additionally, ensure it is refined enough to be compatible with your software.
But most importantly, have something in the dataset that will help you find the answers you seek.
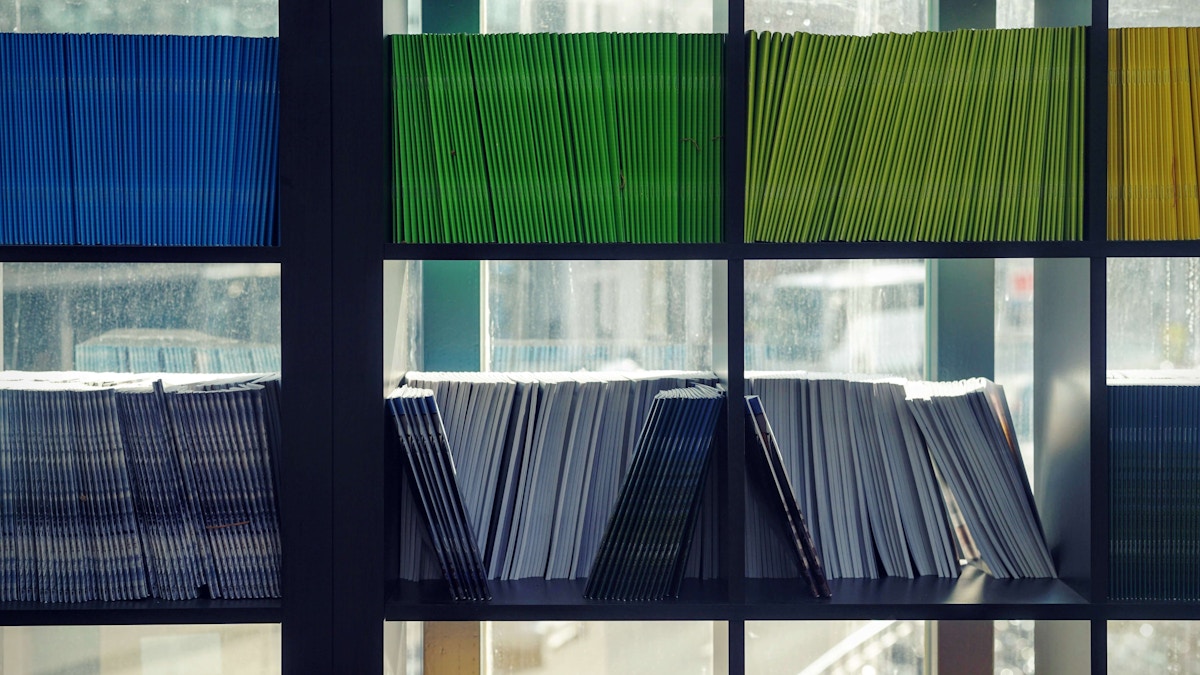
Why should businesses use refined data instead of raw?
The main reason for using refined data instead of raw is reduced time to value. Instead of going through all the data refinement steps listed above, your data team can start analyzing much faster if you get a ready-made refined dataset from a reputable vendor.
Subsequently, you get insights before the market changes, thus outpacing the competition.
Of course, there’s more to that. Refined data means a lightweight database that can be up to many times smaller. This way, you save precious storage space and time by being able to process it faster.
Last but not least, such data is also ready for enrichment, discussed in the section below.
Refined data enrichment
If you thought refined data was the highest form of information, think again. Currently, this title belongs to enriched or enhanced data.
Such a dataset has already been filtered, standardized, and refined. Plus, it includes extra or missing information from other sources, such as a second database you bought or created yourself. This usually comes in the form of additional data points or records.
Let’s say you want to invest in new businesses and acquire a startup database. This database includes founders’ names, headcounts, and founding years, among other things. Now, combine that with the last funding date, type, and amount raised from the company funding dataset, and you’ve got yourself a much stronger profile.
In a nutshell, refined data enrichment allows you to dig even deeper and have a truly holistic point of view.
Where to buy refined B2B data?
The supply of refined B2B data still cannot compare to that of the raw version, which has long been the sole option. However, we see clear signals of a growing demand. More and more companies want actionable, ready-to-use data, and the advent of AI will only make raw data refinement easier.
At the moment, refined B2B data is offered in multiple data marketplaces under different names, including clean, cleaned, and filtered, to name but a few. Therefore, before buying, you should learn what stage of the refinement process their product is in.
Another source of refined data you doing your own scraping. However, this requires knowledge and extra resources that smaller companies often lack. So, if you’re not already scraping, you should probably choose one of the other two options.
Finally, the last option to get refined data is buying it straight from the data provider, such as Coresignal. This way, you can avoid any fees that data platforms might charge or higher prices because the platform charges the seller.
Don’t miss a thing
Subscribe to our monthly newsletter to learn how you can grow your business with public web data.
By providing your email address you agree to receive newsletters from Coresignal. For more information about your data processing, please take a look at our Privacy Policy.
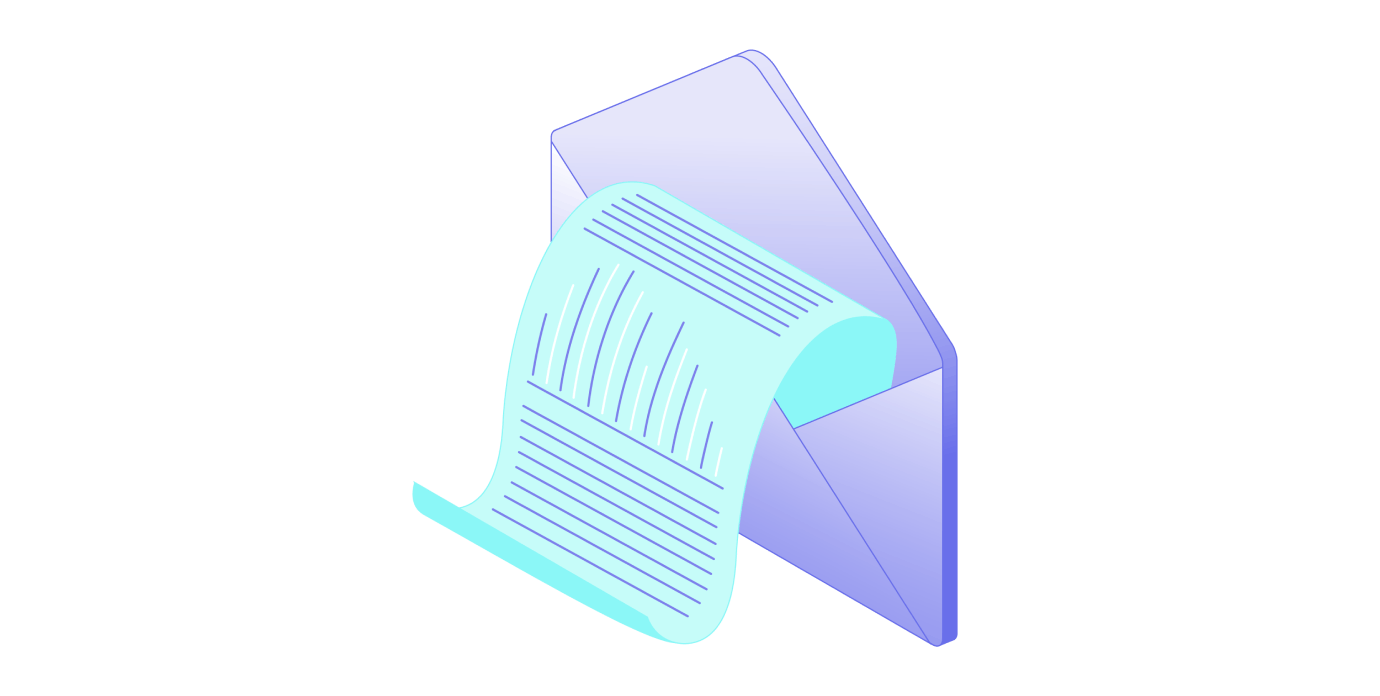
Related articles
Sales & Marketing
10 Most Reliable B2C and B2B Lead Generation Databases
Not all lead databases are created equal. Some are better than others, and knowing how to pick the right one is key. A superior...
Mindaugas Jancis
April 23, 2024
Sales & Marketing
It’s a (Data) Match! Data Matching as a Business Value
With the amount of business data growing, more and more options to categorize it appear, resulting in many datasets....
Mindaugas Jancis
April 9, 2024
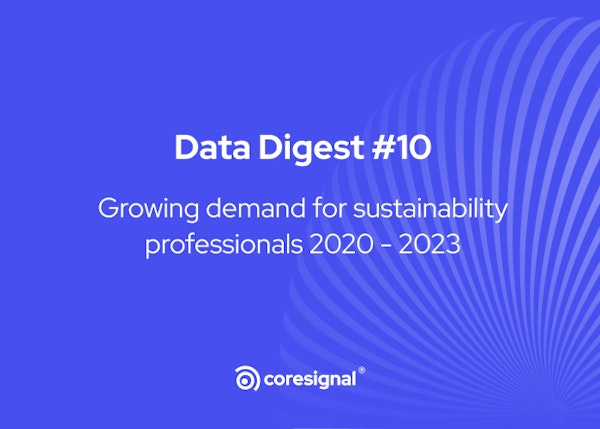
Data Analysis
Growing demand for sustainability professionals 2020 - 2023
Original research about the changes in demand for sustainability specialists throughout 2020-2023....
Coresignal
March 29, 2024