All data-driven businesses that build products or generate insights using external data know that working with incomplete or inaccurate information leads to unreliable results. Buying data that has already gone through some data cleaning saves time but comes with challenges.
In this article, I'll discuss why web data cleaning differs from cleaning other types of data and share some tips for businesses based on my experience in the web data industry.
Defining clean web data
Public web data is any data that can be accessed publicly online. When scraping web data, companies work with unstructured, raw data that needs to be parsed and go through a certain level of processing to make it more structured and readable for such tasks as analysis or machine learning.
However, this processing level still needs to be improved for most analysis use cases as, at that point, data still contains duplicates, fakes, unstandardized values, empty values, and lots of useless data.
Useless data is poor-quality data that is irrelevant in the context of your work. Fake data is also useless data. In this article, we're referring to fake data as data that's not created by humans or is created by humans, but the information in it is not authentic and doesn't create any value.
As a business, you don't want to make decisions based on partially inaccurate, incomplete, and not normalized data. That's why the "garbage in, garbage out" principle is so important. Web data cleaning improves data quality and reduces dataset size, which saves a significant amount of engineering resources and reduces time to value.
Similarly to how a raw photograph file contains all the visual information that a camera absorbs during the brief moment it takes to capture a picture, raw data includes all information in the source.
Clean data is the final photograph the photographer sends to a client. It's the same picture, but the colors are balanced, irrelevant objects are removed, and the photographer may have applied a photo filter they like.
To put it into perspective, I recently worked on a clean dataset that consists of information about companies across the globe. The original dataset contains over 68 million data records. The cleaned version contains almost 35 million records.
It means that almost half of the data records were removed. However, 35 million complete and accurate data records about companies is still a huge dataset that can power products and in-depth analysis.
Web data cleaning examples
Teams that are about to start working with raw public web data should be ready to deal with questions like:
- Where will you get the data?
- What will your data pipeline look like?
- What do you want to do with primarily empty records? Do you want to keep them?
- What if your data has fake records? How do you deal with them?
- How will you tell if, for example, a company profile is not fake?
Freshly scraped data can have various issues, and it takes several data processing steps to turn scraped data into clean data—one of the key ones being date parsing. To make things easier, I'll primarily focus on company data.
Let's take data that reflects companies' founding dates as an example. There are over 20 date formats. Some of them are common, and others aren't. Imagine that you scraped the internet and have data with multiple different date formats: "November 1st, 2023", "2023-11-01", "11/01/2023".
What you want to do is to convert them into one by standardizing the date field. It is complex if you account for human spelling errors and other issues. However, this alone would make actions like filtering much easier.
Here's another example. You scrape web data that contains text fields. You can find additional symbols in the text, like emojis or web links. And various text formatting artifacts that the scraper sees as HTML tags. All these things might make data less meaningful to you.
At last, let's take addresses. Again, you will encounter a variety of versions of addresses, and yet, for most use cases, you will need a unified address format.
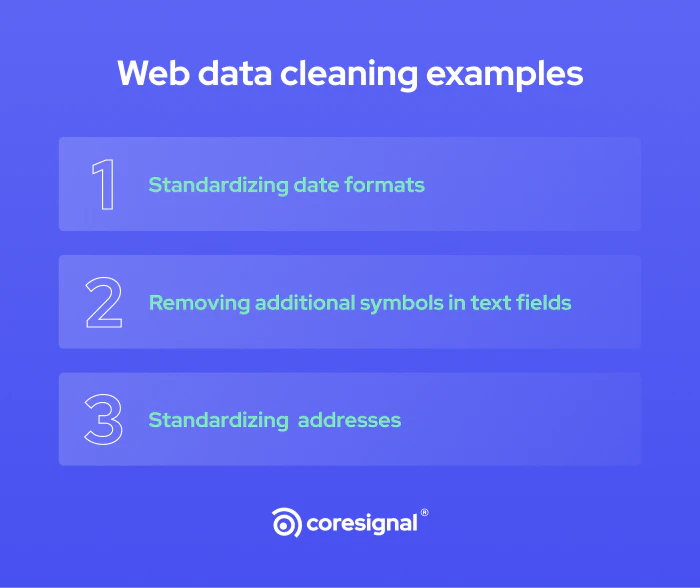
Web data cleaning challenges
You may wonder how web data cleaning differs from other types of data. Well, it's important to note that "web data" broadly describes data in different formats, units, and types. It ranges from free user-generated textual input to pictures, videos, and more advanced forms of textual and other fields with input proofing.
Although the initial description of web data cleaning sounds more or less straightforward, this process has numerous challenges.
- First and foremost, B2B web data is usually big data. Datasets vary from gigabytes to terabytes and more. So, all challenges related to big data processing, such as storing and accessing the data or aligning the pace of your business with your technical capabilities, apply to web data cleaning.
- Second, you will most likely aim to work with structured data without any anomalies. Both parsing and getting a specific piece of information out of parsed data takes a lot of work.
- Lastly, a big part of web data cleaning is proofing. It starts from a theoretical standpoint rather than the actual cleaning. For example, how will you tell the difference between good and fake data? Dealing with fake data is challenging. You need to decide on an approach, implement it, test your thesis, and revisit this question regularly because web data is prone to changes.
Raw or clean data: Which one to buy?
And here, the two worlds collide. Remember how, earlier in the article, I used the phrase "garbage in, garbage out" to describe the parts of data that get removed as not valuable during the cleaning process? Special characters, incomplete values, fake data, etc.?
Ironically, there is another saying about garbage that's very relevant in this situation: one man's trash is another man's treasure. The "impurities" in web data are one of the key things that make some companies want to use it. Here's a couple of examples why:
- The company finds value in HTML tags, control values, unstandardized input, emojis, and similar elements in data that often get removed;
- The company is technically capable and ready to process raw data;
- The company strictly wants in-house data processing: they want to do everything from scratch throughout the whole data lifecycle after collection without any input from other parties.
Although working with raw web data is challenging, such data has immense potential. It's a blank page that companies often need. By opting for raw data, they process it based on their exact needs. They are the ones that give data meaning, both data that they decide to keep and data that they remove or alter.
Clean data, depending on the level of processing that was done, already has some meaning. For example, specific types of input or data records, such as special symbols or incomplete records, were considered irrelevant based on criteria decided by the data vendor.
If your approach aligns with the approach of the data vendor, you will save a significant amount of time and money by opting for already-cleansed data. Clean data is ready for analytical processes and insights generation, eventually leading to a shorter value time.
What mistakes do companies make when purchasing clean data?
Purchasing ready-to-use datasets helps businesses save a lot of time and money that would be spent on collecting and processing web data. Still, when buying clean data, I recommend considering a few things.
A relatively obvious yet possible mistake that you want to avoid when purchasing clean datasets is not knowing what data cleaning processes were actually performed.
- Before buying, ask your data provider the same questions you'd ask yourself if you were to start gathering and cleaning web data: what sources are they using, how do they tell if data is fake or not, what data is removed, what data is altered, etc.
- If you're generating insights that will inform your business decisions or if you're aiming to solve a business problem, you will most likely need more than a single dataset. The same applies to any data purchase.
- Lastly, don't put all the eggs in one basket. Your data team should use various sources and datasets that complement each other to support findings. That is especially true in a company data market, as additional information about companies you're analyzing makes it possible to get unique and reliable insights.
Final thoughts
Data cleaning is a complex and resource-consuming process. Finding a data vendor that can clean and prepare datasets for analysis in line with what you are looking for can be considered a good way to outsource a part of the engineering work.
Although this option is unsuitable for companies that want completely raw datasets, in my experience, many others will benefit from reduced dataset sizes and the opportunity to extract value from data faster.