Public web data can transform your business. It provides unique opportunities and insights. However, a distinct aspect of this data is that it’s raw and, to put it simply, big. Working with large amounts of raw data requires specific strategy, tools, and skills.
In this article, I will be focusing on how to prepare for working with raw web data and how to use it to achieve optimal results. I will cover the first steps of getting started with web data, discuss what resources are needed, and share some useful tips to help your organization do it efficiently.
What is public web data?
In the broadest description, web data is any data collected from the web. It can be data on companies, job postings, reviews, etc. In its raw format, this data comes in files consisting of large quantities of numbers, text and symbols. The term raw data can mean both unstructured and unparsed or fully parsed data that is not cleansed for a specific purpose, depending on the context.
In this article, I will be referring to public web data or public web data as large amounts of raw data collected from public web sources.
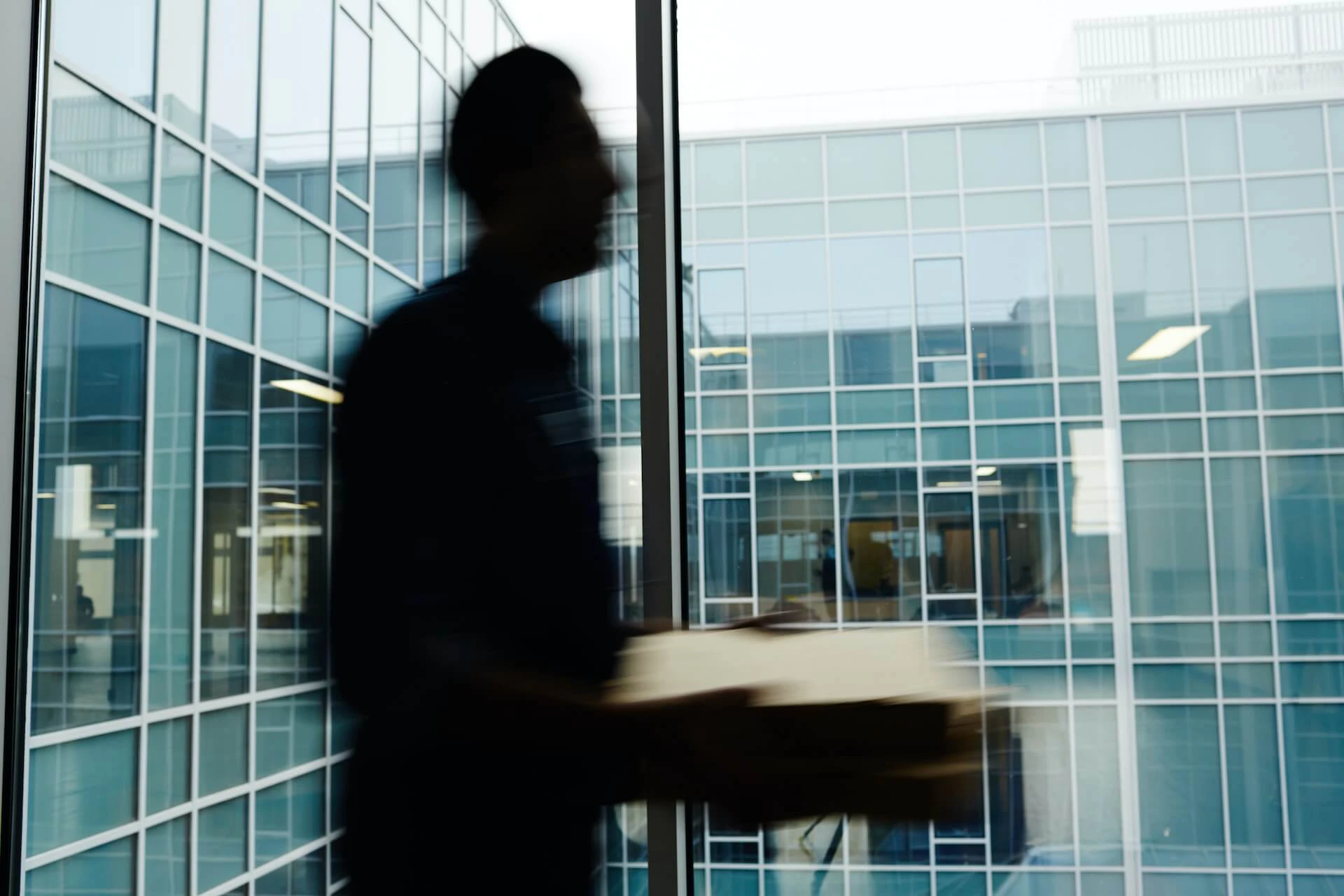
Public web data sources
Public web data can be collected from professional networks, business information platforms, publicly available online databases and similar sources. Collecting public web data gives an public web purpose for many valuable data points extracted from public company profiles , job postings, company or product reviews, etc.
For example, these profiles have company headcount numbers, job descriptions, job titles, product ratings, job requirements, and more. Every field of information on a public web page can provide some value for specific purposes.
What is public web data used for?
Companies mainly use public web data for two purposes:
Business insights. For example:
- Sales intelligence;
- Investment opportunities;
- Monitoring companies they are interested in.
In this case, the company might be looking to use data for business insights, such as finding new startups to invest in or potential clients.
Another example would be monitoring how the competitors are growing their teams. Insights like these are used for internal purposes.
New products (various types of products based on specific data or topics).
In this case, the company is looking to build a data-driven platform or product. They use proprietary methods and AI-driven models to process the data to extract information and insights or enrich existing data and offer it to clients via a convenient and user-friendly interface.
For example, the business provides talent sourcing services in the healthcare industry and uses public web data to build a platform for employers.

Getting started: The first steps and resources
The organization needs to have clear business objectives and data strategy to get started with public web data. In other words, the company has to know what the data is needed for.
This idea should be in the hands of someone who keeps their finger on the pulse of the market, can identify trends, and, most importantly, business opportunities. It will probably be someone in a leadership position. This knowledge and understanding should be the base of the search for data that can be leveraged to achieve your business objectives.
The usage of web data can simply be called signal generation. A company identifies what signals would be valuable for the business, whatever they would be, and searches for data that can help generate these signals.
So, let’s say you already have a business goal or assumption that requires data. The next important step is getting the necessary resources, starting with the data itself.
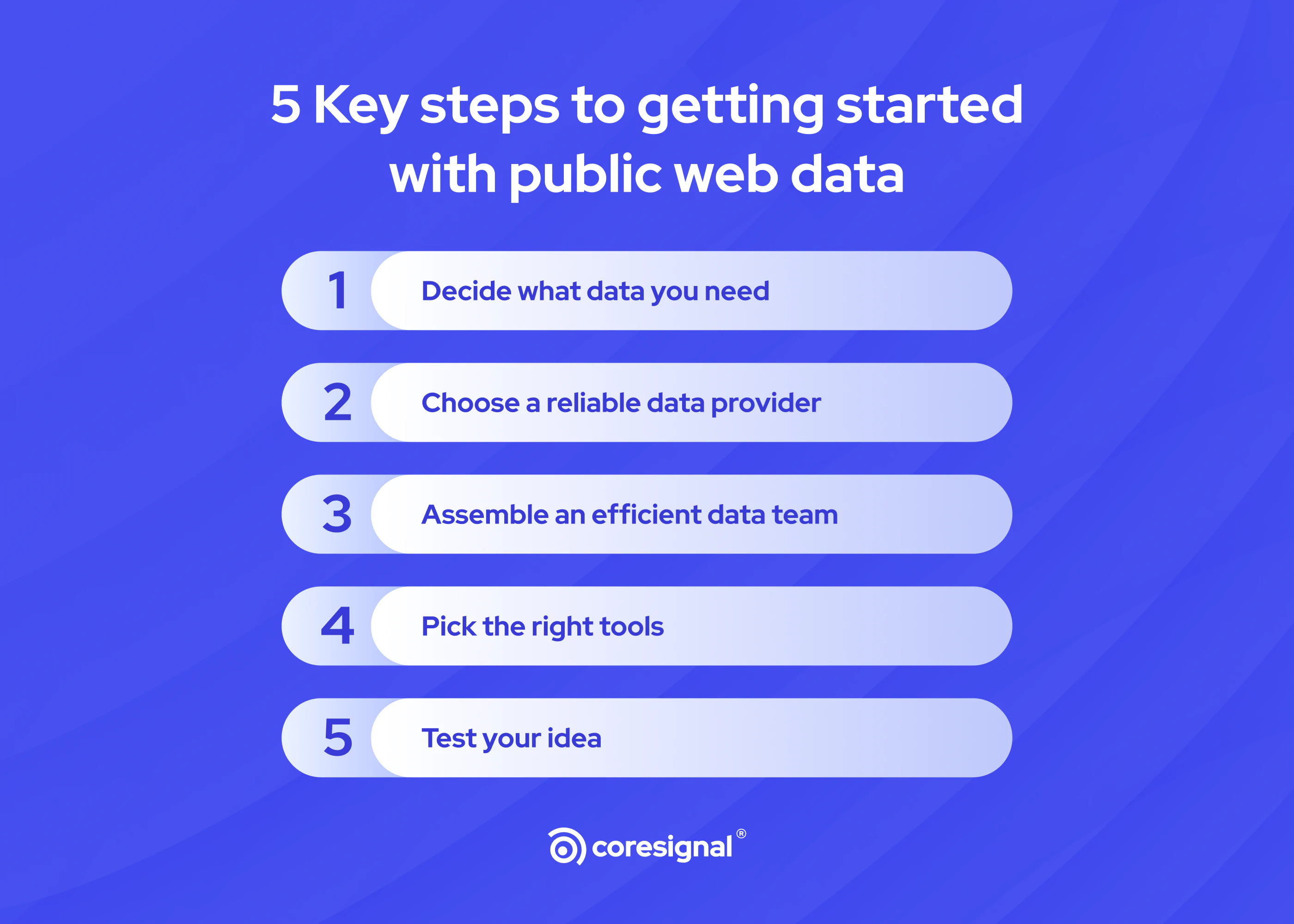
Data
When a company decides that they need to get large amounts of data from an external vendor, the first step is to find a reliable data provider. This search has specific requirements regarding data quality and reliability.
Choosing a data provider
To evaluate data quality, look at such factors as accuracy, completeness, uniformity, and timeliness.
I asked Coresignal’s data experts Martynas Simanauskas and Justas Gratulevicius, who consult businesses on how to leverage public web data for various use cases, to share their insights and to explain why it’s also essential to ensure that the data you’re getting is fresh and its delivery is consistent.
“Data tends to age and lose its relevance very quickly. Coverage is important, but so is freshness. Continuous dataset updates are challenging for data providers, but it provides maximum value for the businesses using this data. It also shows the commitment and expertise level of the data provider,” Martynas explained.
Using a set of public web data only once is quite rare. That’s why, according to Justas Gratulevicius, you should choose data providers that can ensure consistent updates without fail.
“If data delivery gets disrupted for some reason, the process your company has built based on this data would also be disrupted. What’s worse is that it would be nearly impossible to quickly find a replacement for the exact data you were getting. Be sure to choose an experienced and reliable data provider to avoid such risks,” Justas recommended.
From the technical perspective, collecting public web data is hard, especially from some sources. What makes it even more complicated is that data providers must always be ready to adapt to changes like new legal requirements or technical challenges.
Lastly, my recommendation is to keep in mind that it’s essential to test if data matches the business objective before you build entire operations based on it.
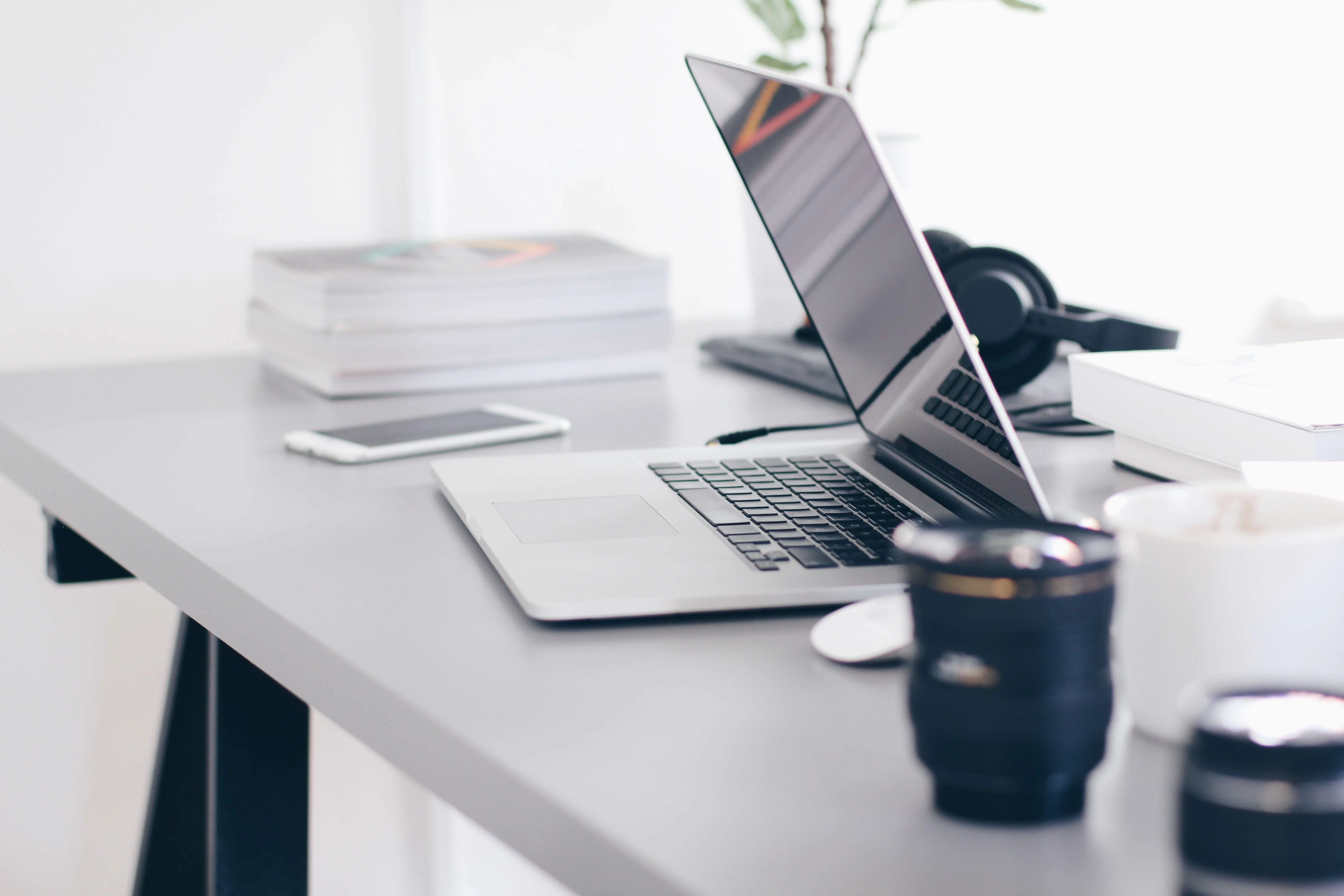
Technology and human resources
When it comes to technology and human resources required for working with public web data, this part heavily depends on what the data will be used for: business insights or building new products.
Data team for simple insights
Suppose the company wants data to generate simple insights for internal use. For example, an investment company wants to monitor startups they are interested in to support their investment decisions.
They need actionable insights, and they don’t necessarily have a preference on how these insights should be presented to them, either via email, during a meeting or in any other format.
In that case, it is possible to build a workflow with one multi-skilled data analyst or data engineer with a few years of experience working with big data.
It might take one to three months to figure out the proper framework for the exact use case. Still, at least one dedicated data specialist will be able to ensure that the data is loaded and aggregated correctly and extract insights from it.
Data team for more complex analysis
If the company is looking for more complex insights, or if these insights will need to be reflected in detailed dashboards or websites (for internal use), more people will be needed. For example, the company wants a continuously updated dashboard based on web data, which the team can access, filter the information in it, etc.
When the project's scope is more extensive, it's better to split different tasks among multiple specialists.
In that case, the data team should consist of a data analyst, data engineer, data scientist, and someone who manages the team.
Team for building new products
Suppose the company is planning to build new products based on public web data. In that case, the team will create a user-facing platform, website, or app, so you need to add front-end, back-end developers, marketing and design specialists, and managers or team leads.
Required skills
From the technical perspective, raw public web data is big data, so your team should have experience working with it or be very keen to gain this skill.
I'm talking about terabytes of data delivered to you regularly, which means that your team should be able to work with specific tools and frameworks, such as the Apache Spark or Airflow, the workflow management tool for big data pipelines.
With the help of suitable frameworks, you can store, load, aggregate, clean, transform data, and perform other actions more efficiently and precisely.
For example, some frameworks allow you to schedule data processing tasks, so there’s no need to wait for some parts of the process to finish before you can move on to other tasks.
And lastly, patience. It might seem obvious, but processing big data is difficult and time-consuming. The amount of data you’re working with can initially seem very overwhelming.
You must be attentive, carefully review everything, keep an eye on any unwanted or incorrect items in data, cleanse the data from duplicates or outliers, and do other things that sometimes cannot be fully automated.
And even when everything is automated, remember that data processing takes days, depending on dataset size and the tools you’re using.

Tools
The first decision you need to make before receiving your first data delivery should be regarding its storage. You can store data in on-site servers or a cloud, which is a more convenient option.
Remember that storing data that you don’t use in the cloud will result in unnecessary costs, so you also need to decide if you need raw historical data. The simple rule is that you will likely need it if you are unsure.
There are options to store old data in a different database that might not be accessible as quickly, and because of that, it will cost you less. There is also the option of only storing the aggregated data, which will need less space.
In every use case, the goal of processing raw data will be to reduce it in size by cleaning or filtering it, which means that the file can transform from terabytes to megabytes.
Regarding data processing frameworks, there are various tools and practices for working with public web data. Here are some examples:
- Hive. Easy-to-use data warehouse system used to analyze large-scale data;
- Flink. A processing engine used for processing large-scale data streams and analytics;
- Storm. A big data processing engine for quick processing of high-velocity data;
- Hadoop. An open-source batch processing system that can be utilized to process big data sets and distributed storage;
- Spark. Tool used for data processing, cleansing and transforming.
Your technical team (or data team) can decide which tools suit their needs and skills. Data providers also offer various solutions which you can pick based on your use case, for example, application programming interfaces, which allow you to access and retrieve fresh data on-demand.
Final thoughts
Your data strategy starts with a vision. When you decide what signals you're expecting to get for your business, it will be easier to choose the correct data and data provider and build a team that will help your business succeed.
If you need help getting started, don't hesitate to contact our data experts!