Predictive modeling plays a critical role in the modern-day digital business landscape. As a subset of AI, it helps business organizations tap into the power of predictive modeling techniques, predictive analytics, and current and historical data to predict future outcomes, identify investment opportunities, and anticipate risks.
Thanks to the power of AI and machine learning predictive modeling, companies can build predictive analytics models to forecast future events and trends and boost business growth. Data analytics provide accurate and up-to-date insights into your competition, target audience, and business operations.
Feeding your forecast the right data points can help your organization improve every aspect of business-related operations. Today, we’ll delve deeper into predictive modeling to explain what it is and how it can benefit your business. In addition, we’ll mention the best places to look for sample data for your predictive modeling algorithms.
What is predictive modeling?

Predictive modeling uses data analytics and machine learning to forecast future events from available data. It involves creating and continuously updating a statistical model that provides insights and helps make data-driven business decisions. Predictive modeling is the main part of predictive analytics, which also deals with managing risks, detecting fraud, analyzing customer behavior, and enhancing efficiency.
For example, you can forecast the outcome of your future marketing campaigns or use popular predictive analytics models to empower your data scientists to develop a unique statistical technique based on historical data.
Predictive techniques rely on input data and common predictive algorithms to forecast trends, behavior, and activity across markets and industries. Aside from predicting the future, predictive analytics tools can also forecast outcomes.
Common predictive algorithms are vital elements of predictive analytics that rely on current and past data to predict an array of business-related applications, such as market trends, consumer preferences, and competitor activity.
You can use all available types of data to train your predictive model-building efforts, such as:
- New data on economics
- Public web data
- Relevant data on your target audience
- Customer service data
- Advertising data
- CRM data
- Transactional data
You can rely on predictive machine learning models to analyze historical data and tackle challenges such as overcoming technical and organizational barriers. Predictive modeling is vital to gaining a competitive advantage, increasing profits, and improving decision-making.
Benefits of predictive modeling

Using predictive modeling and analytics in your organization can benefit your business in various ways. For example, predictive models excel at detecting fraud. You can identify and mitigate fraud attempts before they escalate beyond repair.
Thanks to the immense power of predictive modeling, you can shield your operations and data from cyber attacks to take your cybersecurity measures to the next level. Many business organizations rely on predictive models and analytics to detect vulnerabilities and anomalies in cybersecurity systems.
On the other hand, predictive modeling is the perfect solution for mitigating collective risk. Predictive modeling can benefit every aspect of your business. For example, you can get an accurate and up-to-date demographic picture of your target audience, including buying habits, spending preferences, interests, etc.
Whether your business operates in the finance, healthcare, insurance, or IT sectors, predictive modeling can help you optimize your marketing strategy and enhance your campaigns with accurate customer data to forecast future marketing decisions.
Other benefits of predictive modeling include:
- Gaining actionable insights on your competitors
- Reorganizing marketing strategies to reach wider audiences
- Optimizing existing products and services for broader markets
- Understanding your customers and their needs
- Understanding industry and market events and trends
- Reducing cost, effort, and time of predicting outcomes
- Detecting cybersecurity threats and identifying financial risks
- Forecasting external factors that impact operational workflows and productivity
- Streamlining resource and inventory management processes
- Identifying future trends
- Churning analyses
- Planning workforce
Types of predictive models
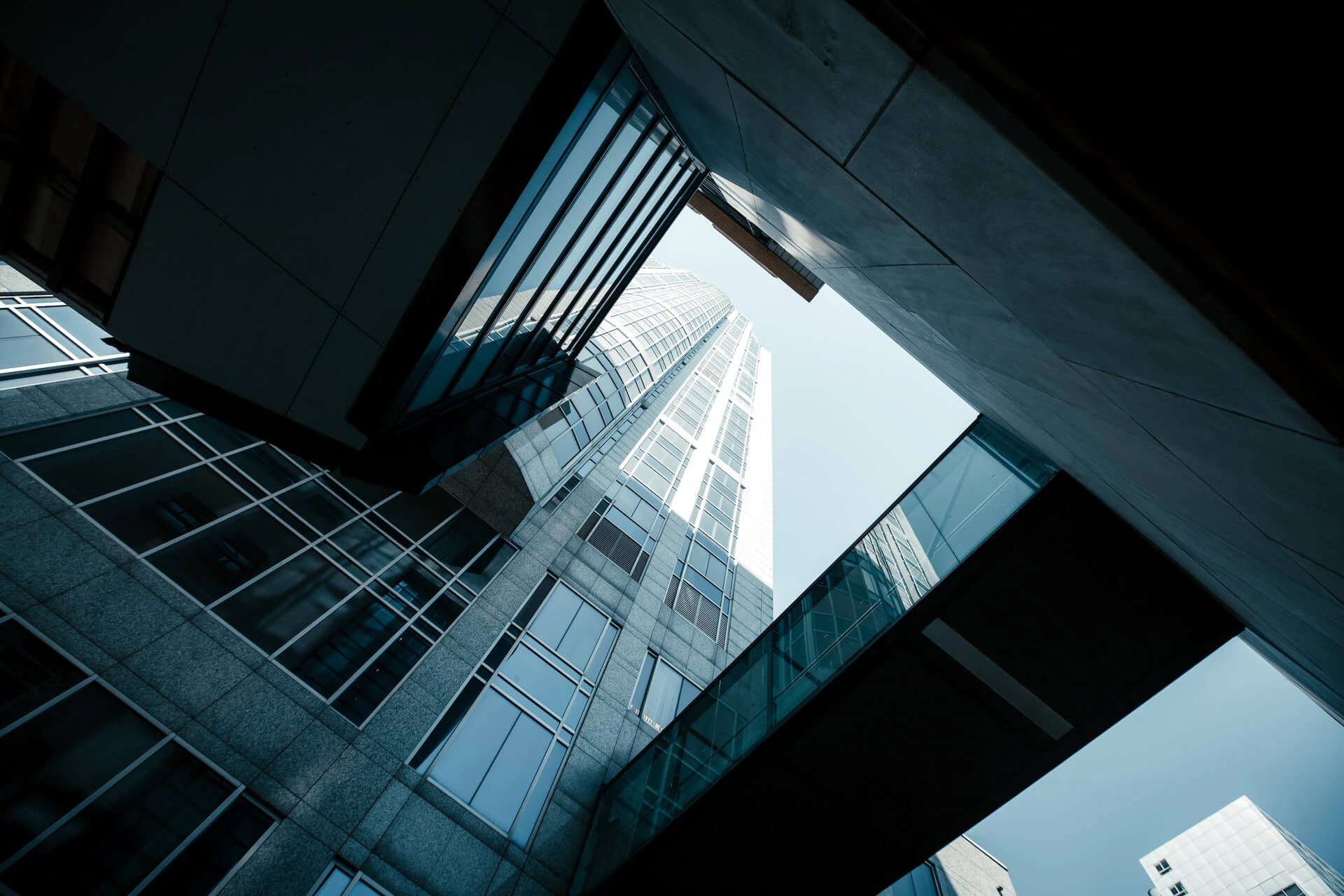
All types of predictive models fall into two categories: parametric and non-parametric. Parametric models base their predictive power on specific parameters, such as discrete numbers.
Conversely, non-parametric predictive models require data from sources that don't fit with specific factors or sets of parameters.
With that in mind, let's explain common predictive modeling types.
Gradient-boosted model
This predictive model generates rankings based on several related decision trees by building one tree at a time. It fixes errors from the first tree and builds upon them to create another enhanced one.
A gradient-boosted model enables you to build several decision trees depending on your needs. This model is perfect for predicting potential search engine results.
Generalized linear model
This model is a powerful statistical tool that analyzes how variables interact to turn variable data into actionable insights. Many data experts see it as integral to statistical processes, such as logistic regression analysis.
Put simply, a general linear model helps your organization make a prediction based on all data in your pipeline by comparing all attributes to find discrepancies and ascertain the accuracy of the prediction made.
Neural networks
A neural network is a complex predictive modeling technique that empowers decision-making processes. It uses algorithm collaboration to recognize patterns, identify data clusters, and categorize them into variable datasets.
Each neural network has three layers:
- Input layer – transports data to the next layer
- Hidden layer – this is where complex functions generate predictors
- Output layer – gathers data from predictors and provides the user with a comprehensive outcome
Neural networks are perfect tools for clustering data without data loss or leaks.
Decision tree
A decision tree is a machine-learning algorithm that gathers multiple data sources and graphs them into a tree-like structure. A decision graph analyzes different decisions and branches them into possible outcomes to match each decision with potential consequences.
Decision trees are ideal for turning organizational data into actionable insights to improve collaboration and ensure every department in your company is on the same page.
Outliers model
An outlier model analyzes datasets to identify outlying or abnormal information within them. That can be useful when ensuring your datasets are accurate and up-to-date across your pipeline.
Outliers models are perfect for detecting fraud and preventing unauthorized access during financial transactions.
Clustering model
Clustering models are superb predictive models for categorizing data. They help you separate data sets and group them based on similar attributes.
A clustering model will take data from each group and cluster it on a large scale to help you assess potential outcomes for each cluster.
This type of predictive model is ideal for custom-tailoring marketing campaigns according to specific demographics.
Forecast model
A forecast model generates numerical outcomes by processing historical data and assessing information based on the given data. This model is ideal for e-commerce websites and online retailers to ascertain customer demand within a set time window.
Classification model
Classification models are commonly used in finance and retail industries to collect and categorize historical data to answer questions that require simple yes/no responses.
A classification model allows for a high level of customization as you can custom-tailor and modify it according to your specific needs.

Predictive modeling use cases
Because predictive modeling can provide accurate and actionable insights relating to your business, market, industry, competitors, and customers, it can help you improve your business in many fields. Let’s briefly touch upon typical real-life business applications.
Enhanced audience targeting
The success of your marketing strategy depends on matching your messaging with the customer’s needs. In other words, your marketing campaigns must target the right audience to drive the desired results.
Predictive modeling allows you to reach your target audience by helping you understand which consumers will likely buy your products and services.
Streamlined buyer journey insights
The buyer journey is an incredibly complex territory prone to constant changes and fluctuations. Navigating such a territory is virtually impossible without a chart.
Predictive modeling acts as a compass that leads your customers further down the sales funnel by offering fragmented experiences to each consumer.
It gives you real-time customer insights to help tailor the buyer journey and customer experience according to consumer expectations.
Accurate investment risk assessment
Predictive modeling excels at predicting investment-associated risks. In addition to harnessing the power of past, current, and future data to help you discover investment opportunities across new markets, predictive models improve your investment efforts by empowering you to recognize lucrative endeavors more efficiently.
You can rely on predictive modeling to forecast investments in particular markets based on historical data to determine how many opportunities can yield acceptable ROI. Assessing and avoiding investment risks is crucial for driving business growth. Thankfully, you can quickly evaluate emerging risks and take adequate mitigation measures to prevent them.
Data acquisition for predictive modeling
You can consider free and premium data sources for predictive modeling. Top free sources for data acquisition for predictive modeling include:
- Datasets for Regression and Classification
- Datasets for Text Mining
- Kaggle Competition
- UCI Machine Learning Repository
- Amazon Public Datasets
- Microsoft Research Repository
- Yahoo Datasets
- AppliedPredictiveModeling R Package
- Machine Learning Data Set Repository
Though these sources offer free data for predictive modeling, no one can guarantee you’ll find what you’re looking for with any of these solutions. If you don’t want to leave anything to chance, consider premium data acquisition sources like Coresignal.
Data providers like Coresignal offer you 24/7 access to a reliable, accurate, and up-to-date public web data for your business needs.
How to use web data for predictive modeling?
Modern consumers are creatures of habit. The reasons that made them buy your products in the past will be the same reasons they will continue to be loyal to your brand. So, you can learn from previous interactions with your brand and leverage that knowledge to enhance your approach toward meeting their future demands.
The key to accomplishing this goal is gathering as much web data on your customers as possible. Predictive modeling with web data requires three vital steps. First, you perform thorough data analysis to determine what worked for your customers in the past and recognize customer patterns.
Then, you use that knowledge to ascertain new patterns your customers will likely follow. Next, you fuel a predictive model with future customer insights to determine which marketing approach worked the best in the past by analyzing different segments of your customer base.
That should tell you which marketing strategy gives the best results for each segment. Finally, you use web data to ascertain which products or services had the most success during each campaign, including the ones that did or did not successfully increase your sales.
Then, you apply those insights to your current marketing campaigns to predict whether those same products or services have a reasonable probability of success if marketed.
Conclusion
Predictive modeling is an excellent way to predict your next business in an overwhelmingly competitive business landscape. That’s why it supports an array of business applications. Though it requires a steep learning curve to get into it, predictive modeling can improve your decision-making, help you avoid risks, and make your business more profitable.
However, accomplishing such goals requires selecting a suitable predictive model for your business niche and acquiring the right data sets to train your model to integrate seamlessly with your ongoing systems and processes.
Thankfully, the web gives you access to a fine selection of free and premium data sources for your consideration.