Public web data is a powerful tool for data-driven companies looking to generate unique business insights or create new products. Acquired from publicly available online sources, web data has various use cases, such as investment intelligence, data-driven recruitment, and lead generation.
The number of businesses using web data is growing, and they all have to deal with similar challenges along the way.
In this article, I will cover the most common web data challenges and share practical tips for overcoming them.
Public web data challenges
Data quality
Let’s start with the cornerstone and the most common challenge of working with public web data, which is quality. Usually, organizations use web data for two purposes, building new products or generating insights. Both of these use cases require accurate and reliable data. Its quality can be measured based on various dimensions, including those described below.

- Accuracy. If data is accurate, it is authentic, correct, and accessible.
- Completeness. Complete data records have all data points with filled values. If some values in a particular dataset are missing, it can distort the analysis results.
- Consistency. Consistent data doesn’t contain any conflicting information or illogical data entries, meaning that the same data matches everywhere you keep it. One of the reasons why inconsistent data occurs is when different users input the data.
- Timeliness. Timeliness means that data is fresh and up-to-date.
- Uniformity. If data is not uniform, some entries have different measurement units, for example fahrenheit and celsius. Uniformity is defined by the consistency of those measurement units.
- Uniqueness. Unique data is original and without duplicates.
I recommend you use these dimensions to evaluate the data you’re working with and to maintain data operations that will consistently ensure you’re using and managing data the best possible way.
What’s also essential is to work with experienced data providers who can provide proof of following the best practices to ensure data quality. Even if it’s not clear before you start working with data from a new provider, eventually, the lack of attention to specific aspects of data quality will become more visible to you once you start testing with it.
The lack of know-how in the company
Working with public web data requires specific resources, including the right talent for the job. But what’s often overlooked is that hiring a data team or technical team capable of processing web data is not enough. You need to know how to analyze your data and how to apply your findings to your strategy, and that involves other teams as well.
According to research, the lack of analytical know-how in the company is the number one challenge of big data implementation. More than half of the companies participating in this research have experienced this issue.
To avoid being in this situation, it’s necessary to examine which stakeholders are involved in working with web data in your company across different teams and levels. Ensure that adequate training resources are available to those who lack specific skills.
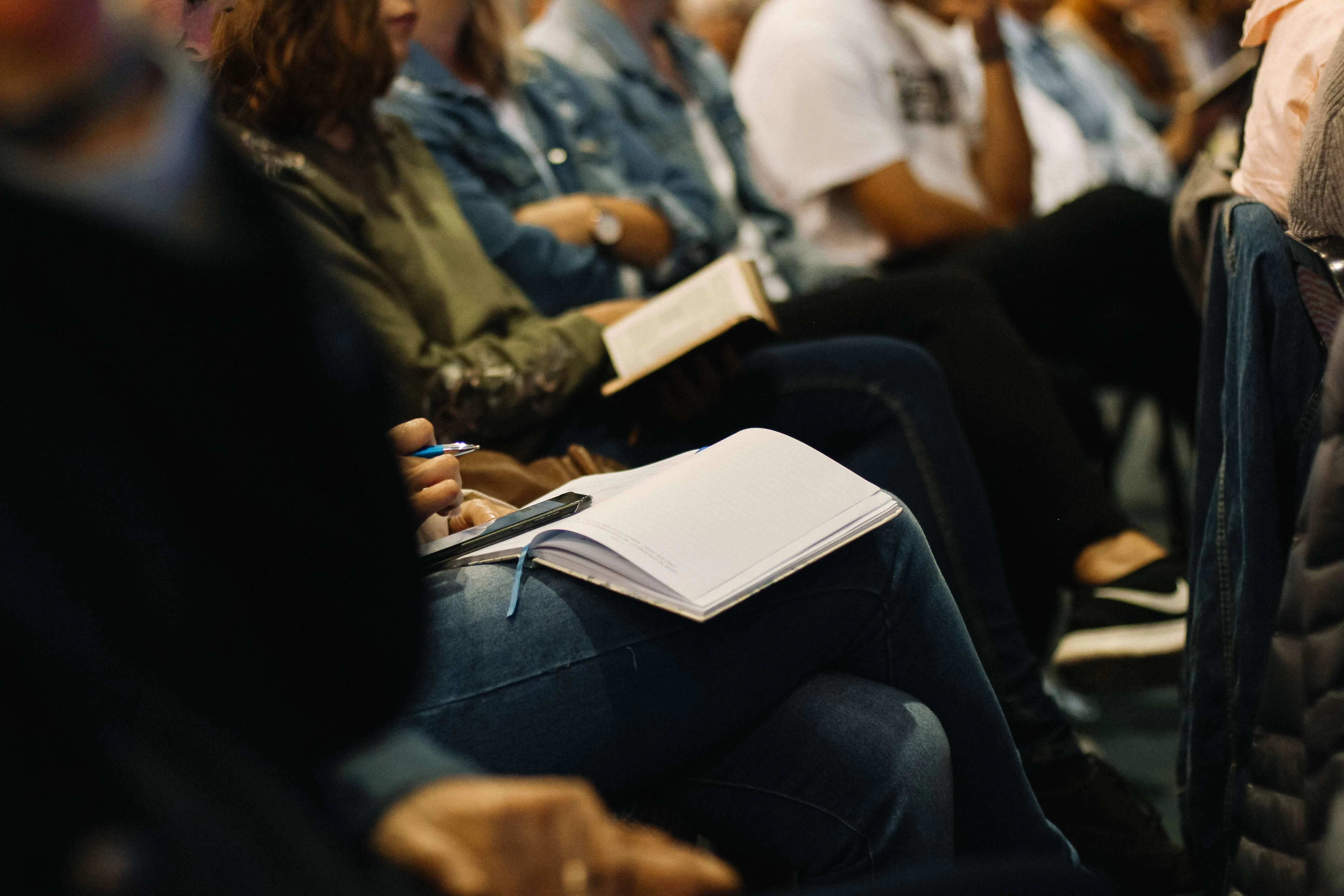
Managing large data volumes
Working with web data requires solutions suitable for managing large amounts of data. I covered the different types of storage solutions in our recent article about preparing for working with web data.
Here are some challenging aspects of managing large amounts of data that I encountered in my experience:
- Storing and accessing historical data
- Finding an efficient way to analyze data
- Aligning the pace of business with the capabilities of your system
Let’s dive into one topic that correlates with every bullet point I mentioned above - technical issues that are inevitably tied to business goals.
When working with big data, one of the most critical things about data management is how quickly your system can process queries. To put it into perspective, a simple query, such as filtering out specific data, can take hours.
Let’s say a company is at the exploratory analysis stage - the data team is trying to filter, calculate and perform other actions with new data to determine how to use it to get the best results. Even this initial analysis might take days because of slow query processing.
Besides quick query processing, another integral part of working with public web data is storage - where and how you are storing data. Various challenges arise simply from how you store your data - where you keep it, how much it costs, how quickly you can access it, and so on.
For a long time, the most common approach in companies was to manage query processing and data storage using a data warehouse system.
A data warehouse is a centralized data system consolidating large amounts of processed data into a consistent, integrated system. Over time, it creates a library of historical data records, which can be retrieved for analysis.
However, there are a few challenges associated with the approach:
- High cost of maintenance
- Slow processes
- Hard to scale
Because of the issues listed above, in the past few years, many businesses working with large amounts of data have decided to transition to a different type of data architecture, which introduces us to two other terms, data lake and data lakehouse.
Data lake is a repository, which is centralized. It allows you to store and process structured and unstructured data in large amounts. The main difference between a data lake and a data warehouse is that the lake consists of separate data storage and processing layers. This separation in data lakes gives companies more flexibility and possibilities to scale their data operations.
The more advanced system that is becoming increasingly popular is data lakehouse. Data lakehouse is a relatively new concept of an open data architecture which combines the cost-efficiency, flexibility and scalability of data lakes and the capabilities of transaction management that a warehouse offers. The different elements of these two systems make data lakehouses reliable, fast, and cost-efficient.
Whether you’re thinking about starting to work with public web data or facing data management challenges with an already existing big data operation, consider exploring the benefits behind the transition from data warehousing to data lakehouse.
Not having historical data
Historical data is valuable for multiple use cases. Here are some examples:
- Building predictive models
- Backtesting and analysis
- Evaluating company performance
However, there are two reasons why historical data could be a challenge. First, finding data providers that offer high-quality historical web data takes time and effort.
Good quality, in this case, refers to complete and accurate data, which means that data should have been collected throughout a specific period without discrepancies.
Secondly, it might be difficult and expensive to maintain large amounts of historical data at your end because of storage, which I discussed earlier.
There are two ways to overcome the problems related to historical data. The easiest option is to work with reliable data providers who offer historical data at request.
The other option is to store historical data yourself. If you decide to store historical data, I found the following practices to be the most effective:
- Only keep the data that you need (without duplicates, irrelevant fields, and other information);
- Store data in small files (processed data instead of raw data, if possible);
- Choose a convenient storage solution that is suitable for your needs of storing and accessing historical data;
- Revisit your decision to store historical data. Time by time, you should audit your internal data-related processes to see if they still match your business goals, including the need for historical data.
No single source of truth
Companies build data models based on raw public web data to extract signals they are looking for, such as investment opportunities or insights into how competitors are doing.
By building a data model based on information like firmographics or data on talent movement across companies, you assume that specific data points signal something significant to your business.
For example, you may assume that the company is growing successfully if the number of job vacancies has increased significantly and the overall number of employees is staying the same.
If you use public web data to extract these insights, it usually means that in its raw format, this data is without any context. So, the result depends on how you process textual or numerical values based on specific filters, how you interpret the data, and so on.
Simply put, you give the meaning to this data and decide what it signifies. That’s why it’s essential to test and confirm that specific signals extracted from data confirm the hypothesis.
All these things can impact what insights you’re getting out of it. This is why it’s crucial to choose suitable sources for your hypothesis, test it, and use those that work for your use case.
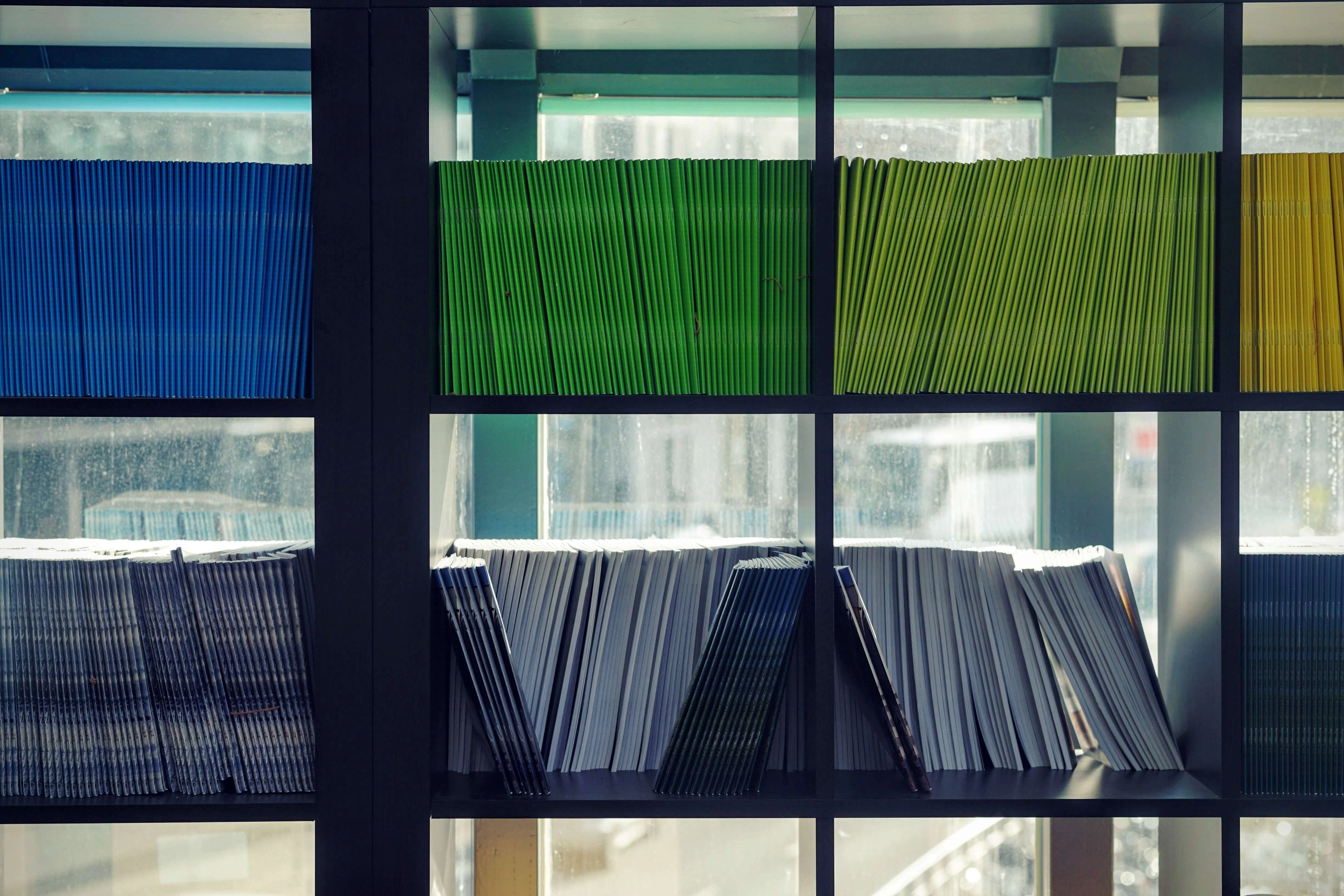
Data on specific industries and regions
Valuable source of public web data is professional networks, such as LinkedIn, that contain information about companies and professionals.
For instance, information about professionals, such as their work experience, education, and skills, is valuable for sourcing specific talent for high-impact job positions, investment research, and competitive monitoring.
However, data from the most popular professional networks still lacks coverage in specific industries, such as those requiring manual labor (construction, service industry, etc.)
At the moment, no equally big or popular source like LinkedIn has such a large amount of public web data covering these industries. There are some similar sources, but the scale of data they offer differs significantly from data on employees in sectors like tech.
Having millions of fresh data records on employees in these industries would open even more opportunities for talent sourcing, market research, and other use cases.
What’s more, is that each public web data source is more prevalent in a specific region than others. As for LinkedIn, this professional network has members from more than 200 countries, but it’s the most popular in the U.S, which has a population of more than 328 million people. So, around 36 percent of U.S. adults are on LinkedIn.
In comparison, with more than 80 million users, members from India make up the second-largest country audience on LinkedIn.
However, compared to the coverage in the U.S, this number is low, considering that India has a population of more than 1.38 billion people.
The good news is that the number of users on sources like LinkedIn is growing its user base in different regions and countries. Let’s take Singapore as an example. LinkedIn users in Singapore increased by 600,000 in the last three years.
Free text fields
Free text fields complicate public web data analysis because it is hard to build a data analysis model based on user-generated, custom text. In some cases it may not be necessary to use free text fields in data analysis, but they usually make up quite a big part of datasets.
The longer the text field, the harder it is to map predicted input options to extract value. However, it is possible to extract insights based on specific keywords with the help of NLP (natural language processing).
From a technical point of view, NLP adds another layer of complexity to web data analysis. Still, it can also be very beneficial for those who can harness it.
My recommendation here is to add NLP to the list of required skills when planning to build or expand your data and technical teams, as natural language processing is projected to gain even more importance in the future.

Final thoughts
Working with public web data presents some challenges related to different parts of this process, but the benefits outweigh the difficulties.
Although it would be hard to find a universal solution to all these challenges, two things help companies to navigate through them.
First is the attention given to the testing phase, which prevents significant mistakes.
And the second one is being open to reexamining how you work with public web data. Revisiting some of your earlier decisions will help you discover new tools, learn new skills, and optimize your data-related processes. The more we work with large amounts of data, the more we know how to do it efficiently.