Many investors and organizations alike rely on data to enrich their decision-making process. From development to sales, quality data insights can provide professionals with insights into every aspect of their business operations. While this may seem rather straightforward, there are quite a few processes that must be followed so you can utilize data’s full potential. This is where data interpretation comes in.
What is data interpretation?
Ultimately, data interpretation is a data review process that utilizes analysis, evaluation, and visualization to provide in-depth findings to enhance data-driven decision-making. Further, there are many steps involved in data interpretation, as well as different types of data and data analysis processes that influence the larger data interpretation process. This article will explain the different data interpretation methods, the data interpretation process, and its benefits. Firstly, let’s start with an overview of data interpretation and its importance.
Why is data interpretation important?
The importance of data interpretation is not far from the importance of other data processes. Much like implementing data normalization and understanding data quality, proper data interpretation offers real-time solutions and provides more in-depth insights than without it. Particularly, data interpretation can improve data identification, discover hidden correlations between datasets, find data outliers, and even help forecast trends.
Additionally, proper implementation of data interpretation offers immense benefits such as cost efficiency, enhanced decision making, and improved AI predictions. Namely, in a Business Intelligence survey, it was reported that companies that implemented data analysis and interpretation from big data datasets saw a ten percent reduction in costs.
While the importance of data interpretation is undeniable, it is significant to note that this process is no easy feat. To unlock the full potential of your data, you must integrate your data interpretation process into your workflow in its entirety. So what is that process? Let’s take a closer look.
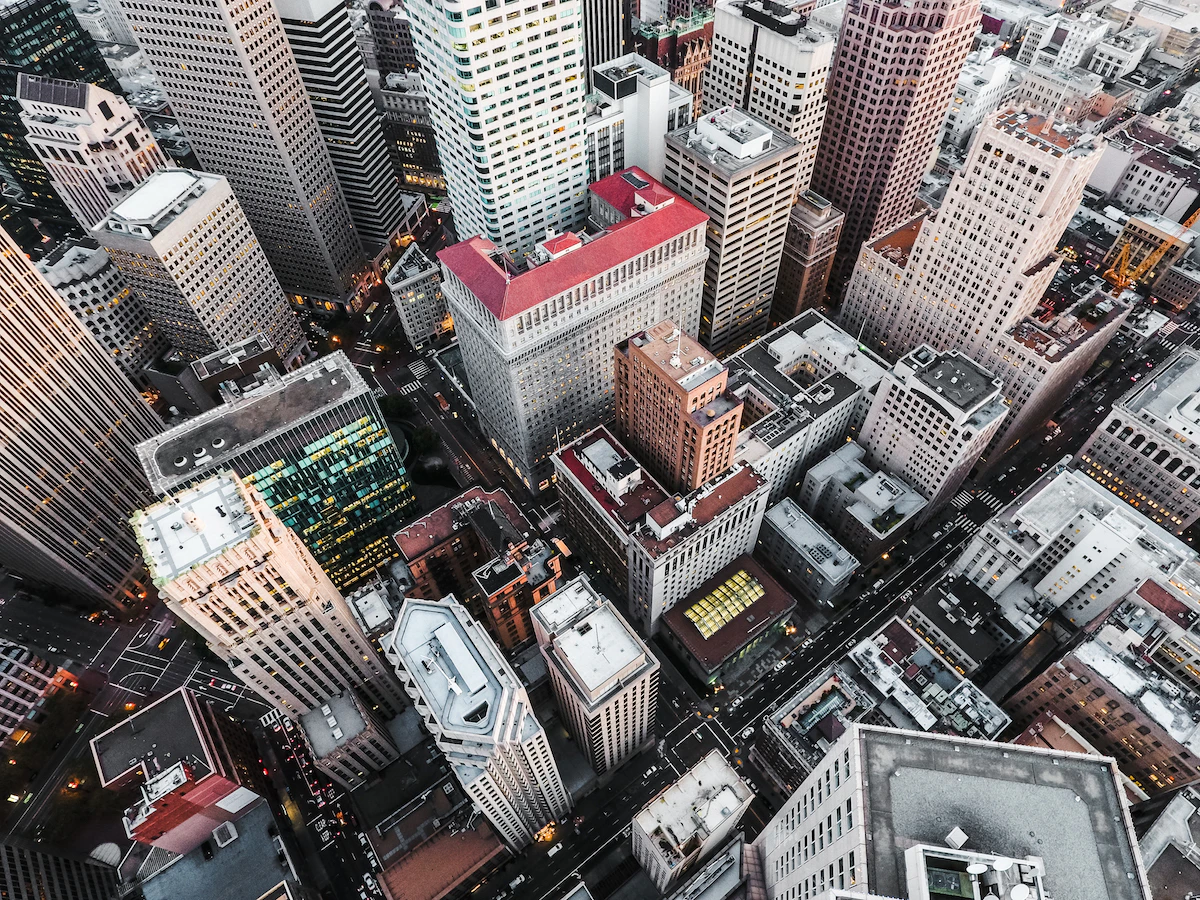
The data interpretation process
Data interpretation is a five-step process, with the primary step being data analysis. Without data analysis, there can be no data interpretation. In addition to its importance, the analysis portion of data interpretation, which will be touched on later on includes two different approaches: qualitative analysis and quantitative analysis.
Qualitative analysis
Qualitative analysis is defined as examining and explaining non-quantifiable data through a subjective lens. Further, in terms of data interpretation, qualitative analysis is the process of analyzing categorical data (data that cannot be represented numerically) while applying a contextual lens. Data that cannot be represented numerically includes information such as observations, documentation, and questionnaires.
Ultimately, this data type is analyzed with a contextual lens that accounts for biases, emotions, behaviors, and more. A company review, for instance, accounts for human sentiment, narrative, and previous behaviors during analysis, helping summarize large amounts of quantitative data for further analysis. Due to the personable nature of qualitative analysis, there are a variety of techniques involved in collecting this data including interviews, questionnaires, and information exchanges. Not unlike many lead generation techniques, companies often offer free resources in exchange for information in the form of qualitative data. In practice, for example, companies offer free quality resources such as e-books in exchange for completing product or demographic surveys.
Quantitative analysis
On the other hand, quantitative analysis refers to the examination and explanation of numerical values through a statistical lens. Similarly, with regard to data interpretation, quantitative analysis involves analyzing numerical data that can be then applied to statistical modeling for predictions.
Typically, this type of analysis involves the collection of massive amounts of numerical data that are then analyzed mathematically to produce more conclusive results such as mean, standard deviation, median, and ratios. Similar to the qualitative process, the collection of this quantitative data can involve a variety of different processes. For example, web scraping is a common extraction technique used to collect public online quantitative and qualitative data. In the same way web scraping can be used to extract quantitative data, such as social sentiment, it can also be used to extract numerical data, such as financial data.
If you're looking for data to identify business opportunities, you can perform both types of analysis with Coresignal's raw data.
How to interpret data
Now that we’ve examined the two types of analysis used in the data interpretation process, we can take a closer look at the interpretation process from beginning to end. The five key steps involved in the larger data interpretation process include baseline establishment, data collection, interpretation (qualitative or quantitative analysis), visualization, and reflection. Let’s take a look at each of these steps.
1. Baseline establishment
Similar to the first step when conducting a competitive analysis, it is important to establish your baseline when conducting data interpretation. This can include setting objectives and outlining long-term and short-term goals that will be directly affected by any actions that result from your data interpretation. For example, investors utilizing data interpretation may want to set goals regarding the ROI of companies they are evaluating. It is important to note that this step also includes the determination of which data type you wish to analyze and interpret.
2. Data collection
Now that a baseline is established and the goals of your data interpretation process are known, you can start collecting data. As previously mentioned, the data collection process includes two major collecting types: web scraping and information exchange. Both of these methods are successful at collecting both qualitative and quantitative data. However, depending on the scope of your data interpretation process, you most likely will only require one method.
For example, if you are looking for specific information within a very particular demographic, you will want to target particular attributes within the larger demographic you are interested in. Particularly, let’s say you want to collect sentiment surrounding an application used by a particular job type; you will want to target individuals with a specific job type attribute and utilize information exchange.
Both of these collection methods can be quite extensive, and for that reason, you may want to enrich your data collection or even fully utilize high-quality data from a data provider. Notably, once your data is collected, you must clean and organize your data before you can proceed to analysis. This can be achieved through data cleansing and data normalization processes.
3. Interpretation (qualitative or quantitative)
This step is arguably the most crucial one in the data interpretation process, and it involves the analysis of the data you’ve collected. This is where your decision to conduct a qualitative or quantitative analysis comes into play.
Qualitative analysis will require you to use a more subjective lens. If you are using AI-based data analysis tools, extensive “coding” will be necessary so that the data can be understood subjectively as sentiment experienced by individuals that cannot be defined numerically.
On the other hand, qualitative analysis requires that the data be analyzed through a numerical and mathematical approach. As previously mentioned, raw numerical data will be analyzed, resulting in mean, standard deviation, and ratios, which can then be analyzed further via statistical modeling to better understand and predict behaviors.
4. Visualization
When your analysis is complete, you can now start to visualize your data and draw insights from various perspectives. Today, many companies have implemented “dashboards” as a part of the visualization stage. Dashboards essentially provide you with quick insights via programmable algorithms. Even without dashboards formatting your data for visualization is relatively straightforward. To do this, you must input and format your data into a format that supports visualization. Some of the more common visualization formats include:
- Barcharts
- Tables
- Scatter plots
- Line graphs
- Pie charts
- Histogram
5. Reflection
Lastly, once you have created adequate visualization types that meet your previously decided objectives, you can reflect. While a rather simple process, relative to the earlier steps, the reflection process can make or break your data interpretation process. During this step, you should reflect on the data analysis process as a whole, look for hidden correlations, AND identify outliers or errors that may have affected your visualization charts (but could have been missed during the data cleansing stage). It is crucial that during this step you differentiate between correlation and causation, identify bias, and take note of any missed insights.

Wrapping up
In all, data interpretation is an extremely important part of data-driven decision-making and should be done regularly as a part of a larger iterative interpretation process. Investors, developers, and sales and acquisition alike can find hidden insights from regularly performed data interpretation. It is what you do with those insights that bring your company success.