Data goes through a lot before turning into valuable insights for an organization. Here is a four-step overview regarding the progression of data ingestion.
- Collecting and ingesting the data;
- Adequately preparing the data through various cleaning and structuring procedures;
- Analyzing and managing the information properly;
- Using it to advance your business goals.
As you can see, the data ingestion layer is the backbone on which all the data-related operations stand. Therefore, it is worthwhile to look closer at this procedure and learn how to improve the data ingestion pipeline for better future results.
Defining data ingestion
It is common to compare data processing and analysis with digestion, adding that before digestion there must be ingestion. So, much like with our bodily function, data ingestion is the process through which the company, hungry for knowledge, gets the information to digest.
Data ingestion is broadly defined as the process of moving data from multiple sources to its correct destination for storage and further usage. This destination is typically a data warehouse, but data lakes can also be used for large volumes of unstructured data.
The data ingestion layer is the initial part of the data pipeline through which a large volume of data is handled and processed for business usage. Data pipelines may include a data query layer where the data is analyzed. Additionally, it can consist of a data visualization layer where it is finally presented for generating insights. As data ingestion is the foundational layer, all the data processing that comes afterwards stands on it, making it crucial to build a high-quality data ingestion pipeline.
Also, it is worth noting that data ingestion should not be confused with ETL (extract, transform, load). Although data ingestion and ETL are related concepts, they are not quite the same thing. ETL is a type of data ingestion process that involves data transformation as its second step. However, data ingestion does not necessarily have to entail such transformation of data.
Types of ingestion
Types of data ingestion can be grouped into three major categories, based on the way data is processed. Each of these types is to be selected based on the data management needs in a particular company and for specific types of information.
Batch ingestion
Batch data ingestion is the type of ingestion where data is collected and sent to the destination in batches at regular intervals. Thus, in batch processing, data is first grouped and held at the ingestion layer until a predetermined time of loading comes. Data might be grouped together based on any kind of predefined logical or structural features. On the other hand, data batches can simply be formed based on the time of the ingestion and pre-decided size of the data batch.
Real-time ingestion
Real-time processing is when the data is collected and loaded straight to the data warehouse almost immediately. Streaming data to the end location always takes a bit of time in itself; therefore, real-time processing happens almost, but not precisely, in real-time. However, extracting data and sending it to the end location happens much faster than with batch ingestion. Consequently, real-time processing is employed when time is of the essence.
Lambda architecture-based ingestion
This is the type of data ingestion pipeline that combines the features of both batch and real-time ingestion. Lambda architecture typically uses stream-processing for online data, and batch ingestion for everything else (e.g., log data). Such data ingestion processes provide a comprehensive view of data at the further layers of analysis. However, this type of data architecture takes more time and effort to build and maintain.
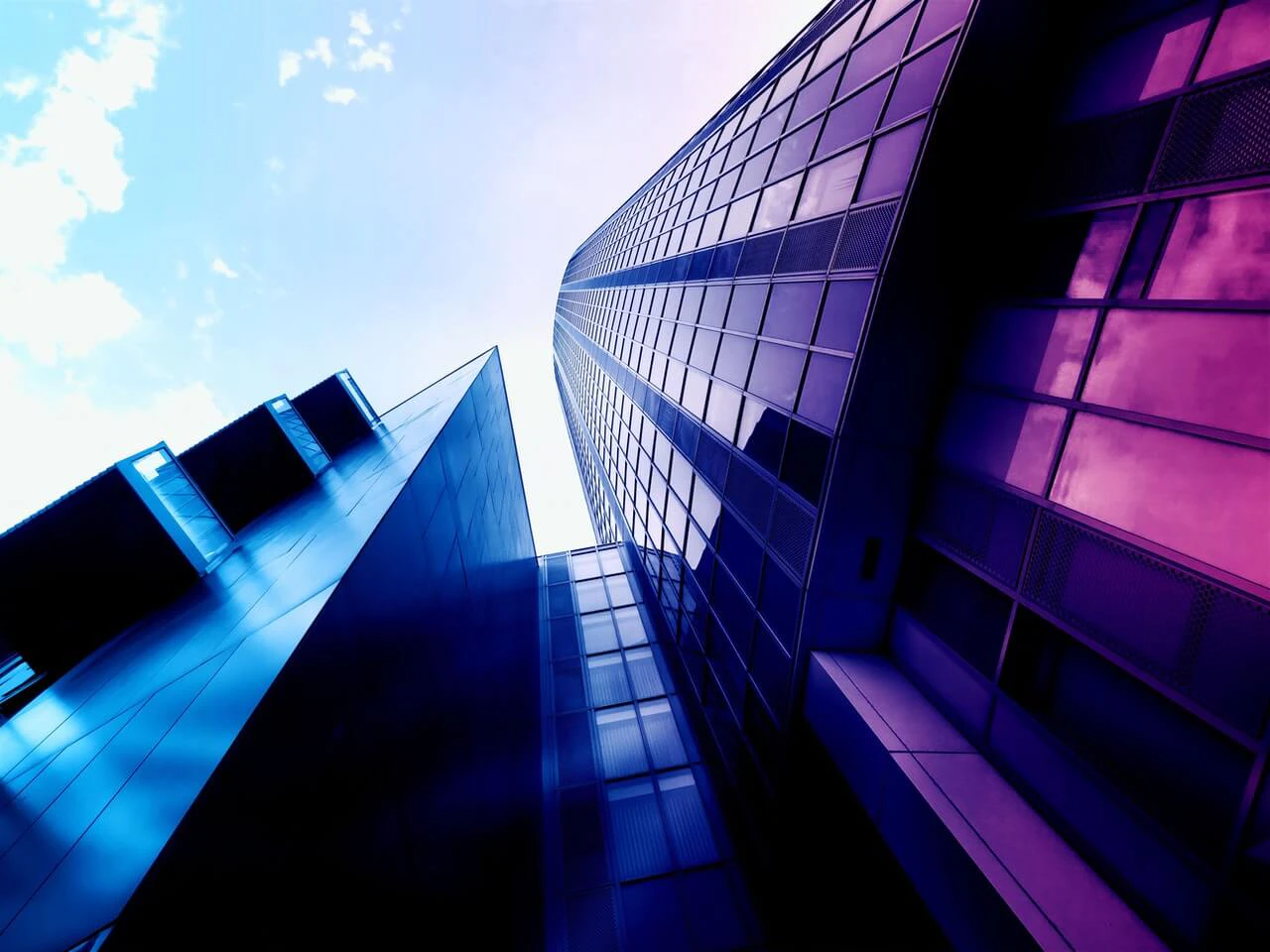
Benefits and use cases
Increased accessibility of data
The main goal of the data ingestion process is moving data from various data sources to its destined data warehouse. Here, data can be accessed by all the users within your organization that need it for their daily work or analysis. Thus, an effective data ingestion process is necessary for making the data available for all those who can benefit the company by using it.
Boosted efficiency of the data-related procedures
The efficiency of all the data-related procedures depends on how fast the data moves through the data ingestion pipeline. Therefore, increased data velocity that comes with an effective data ingestion process makes pretty much all key procedures of business traffic faster. The smoother is the movement of the data, the sooner the business objectives are reached.
Enhanced decision-making
Decision-making in a modern business is highly dependent on business intelligence that comes with high-quality data analysis. But, as mentioned before, you need to ingest the data prior to analyzing and using it. As a result, the quality of decision-making is directly correlated with the quality of the data ingestion pipeline and the procedures that go along with it.
Data consistency
Numerous sources exist that may hold the information that your company needs. This may lead to consistency issues where data from different sources conflict with each other. Well-built data processing system allows removing these issues, creating conditions for further data integration. You can start building it by validating individual files: recognizing and prioritizing data sources with the highest-quality up-to-date data.
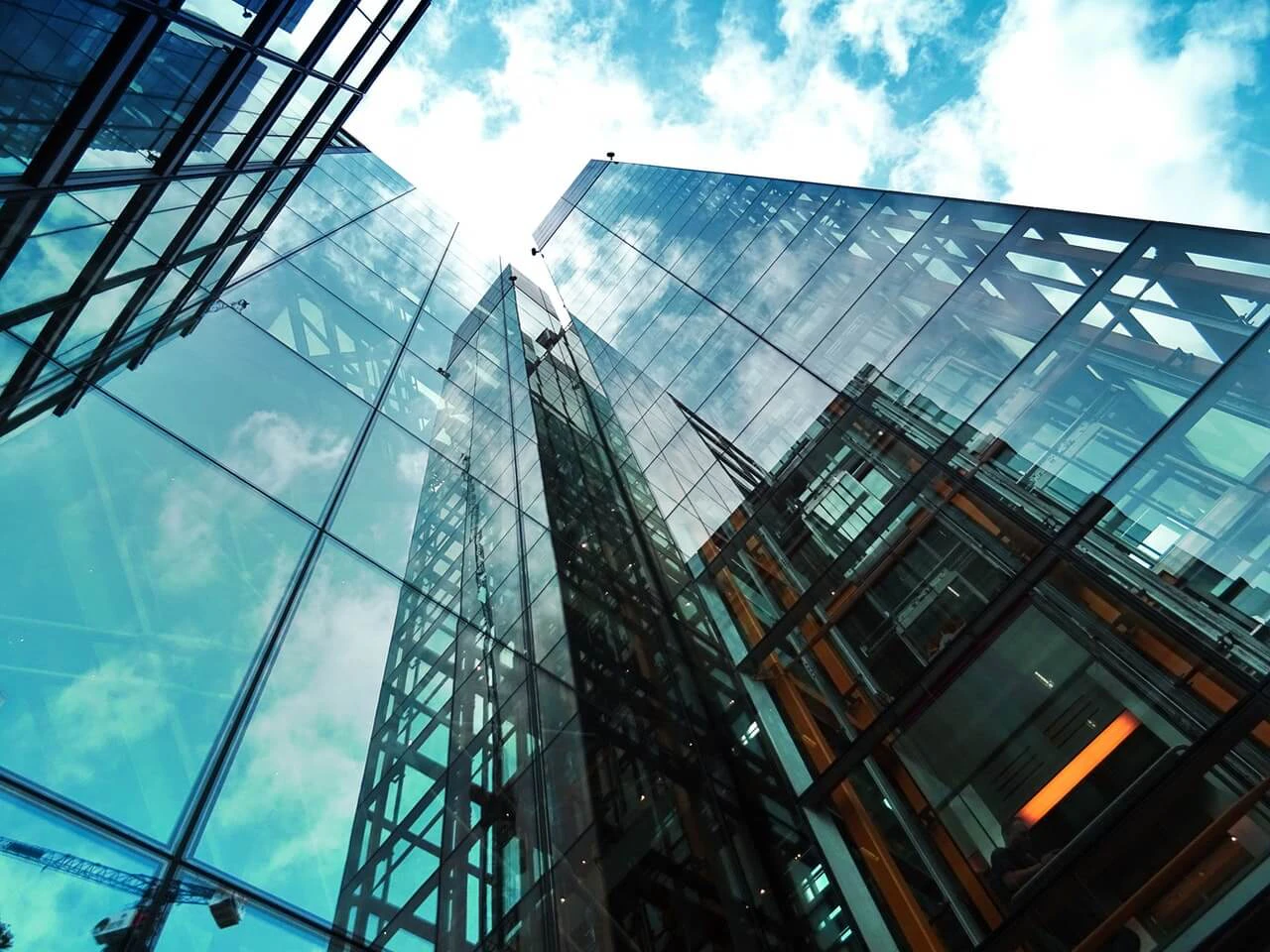
Data ingestion challenges
Update and maintenance take time
One challenge that architects of data pipelines face is that it may take a lot of time to implement the necessary updates on the system. Downtime caused by updates and maintenance issues may be costly to the company; hence, developing new data ingestion methods that would solve these problems is a priority in the field of big data.
Increased data diversity
Data volume has been a problem for quite some time now and businesses were able to achieve adequate results in handling it. Now, many data types and numerous sources exist, creating a new challenge of handling this increased diversity. Moving data from multiple data sources may require constant rebuilding of the data pipeline which, once again, takes valuable time and effort.
Data security risks
Data security is another significant topic you need to consider when extracting data and trafficking it to the data warehouse. This is especially important for widely accessible data storage units, such as the cloud data warehouses. Mitigating these risks takes constant monitoring for the weak spots in the data pipeline, but at the end of the day, secure data brings more value than breached data.
Best practices and selecting the tools
First of all, maintenance issues may always arise with the data ingestion pipelines; therefore, it is advisable to plan ahead and anticipate them. Being prepared for a sudden problem is halfway to solving it. For that reason, backup plans for the downtime of the ingestion of business-critical data should be set and followed when such occurrences happen.
Secondly, when thinking about how to make data-related tasks more efficient, it is always a good idea to consider automation options. The more of the tasks are automated, the more precious time of the employees can be directed where human interference is necessary.
There are many tools that can help automate the handling of the incoming data. When selecting the tool for the particular needs of the company, the basic features of the ingested data should be considered. These include the volume, type, and structure of the data.
Thirdly, time is a key factor when choosing the right tools. Some are meant for real-time processing while others can help with batch data. Of course, user-friendliness, design, and additional features are also important to make sure that the utilization of tools goes smoothly.
At the end of the day, every company is slightly different in its data-handling procedures and objectives; therefore, selecting the right tool is case-specific. One thing that most firms share is the ability to improve their results by proper utilization of such tools.
To wrap up
Data ingestion is the process of getting data to where it needs to be in order to be used effectively and beneficially by the company reps. Hence, making this process as efficient as possible is in the interest of every modern data-driven business.