Data plays a fundamental role in most organizations these days. Companies can't get value from poor-quality data, whether used to build a business strategy or as a base for a new product. Data reliability is an integral part of successful data-driven processes.
This article will explore the basics of data reliability: the key factors, the different ways this term is used in data science, and how to avoid working with unreliable data.
Growing demand for reliable data
As data becomes increasingly important, the demand for high-quality, reliable data also grows. Data reliability is the foundation of data integrity. It strives to ensure that the data you're working with meets specific standards and aims to streamline and optimize data management processes to ensure that data is trustworthy.
As a more general data science term, data reliability refers to consistent and dependable data. When speaking about data reliability, experts usually focus on continuously improving data-related processes in organizations to manage data successfully and ensure its value to users.
In statistics, the term data reliability focuses on data consistency. When data is reliable, it means that if the data collection process were to be repeated, the data would yield consistent results.

Key factors that define reliable data
In essence, reliable data can be trusted to consistently represent what it's intended to capture. Reliable data is trustworthy, accurate, and highly available. Ensuring data reliability requires sophisticated collection methods, validation, and quality assurance processes as it's intended to protect data at all stages of its lifecycle.
Some companies even have dedicated data reliability engineers who address quality and availability issues to ensure the highest level of data reliability.
Major data reliability issues are usually noticed during testing or when stakeholders report data reliability issues. They often arise from quality-related incidents. However, there needs to be a difference between data quality and data reliability.
While quality emphasizes the correctness and usability of the data, reliability is more about whether the data can be consistently reproduced and is dependable. Still, some data quality dimensions are inseparable from data reliability.
5 data quality dimensions
Here are 5 dimensions related to data quality that are also important for data reliability.
Consistency
Consistent data refers to the measure of coherence and uniformity of data across multiple systems. Significantly inconsistent data will contradict itself throughout your datasets and may cause confusion about which data points contain errors.
Accuracy
While accuracy (data being correct and free from errors) and reliability (data being consistent) are not the same, they often go hand in hand. Inaccurate data can sometimes be consistent (and thus reliable), but it will lead to consistently incorrect conclusions. Accurate data is error-free and timely (timeliness is sometimes presented as a separate dimension).
Validity
Data validity refers to whether the data accurately represents what it is meant to measure.
Completeness
Completeness is the dimension that determines the comprehensiveness and wholeness of data, meaning that all needed data is available and no values in data are missing.
Availability
Data availability means that an organization's data is available to its end users and stakeholders whenever they need it.
Trust in data
However, it is understandable that data reliability goes beyond ticking boxes and looking at an exact data file. To build a culture of trust around data, an organization needs to have data teams that strive to ensure data quality, focus on having a shared definition of data across the company, and are also able to build integrity around their work.
When researching, trusting your organization's data, and ensuring data reliability, you may encounter a variety of interconnected terms and goals. That's because it's a process of continuous improvement.
Let's take data observability. Observability defines how a company can track and manage the health of the data it is using.
Trust in data is also related to a more recent term, "data downtime," which is worth looking into. Data downtime aims to show when data quality is bad or data is not available.
Data reliability vs data validity
Data reliability and data validity are often mixed with one another or erroneously used as synonyms because both evaluate the quality of measurement. Data validity focuses on assessing whether data is true, while data reliability checks if data produces expected results consistently.
From this perspective, data validity can be seen as a data reliability component. Data must be reliable to be valid. For example, if you're using data about for-profit companies, but the dataset contains information about non-profits, you're using invalid data. Invalid data will produce invalid results, making it unreliable.
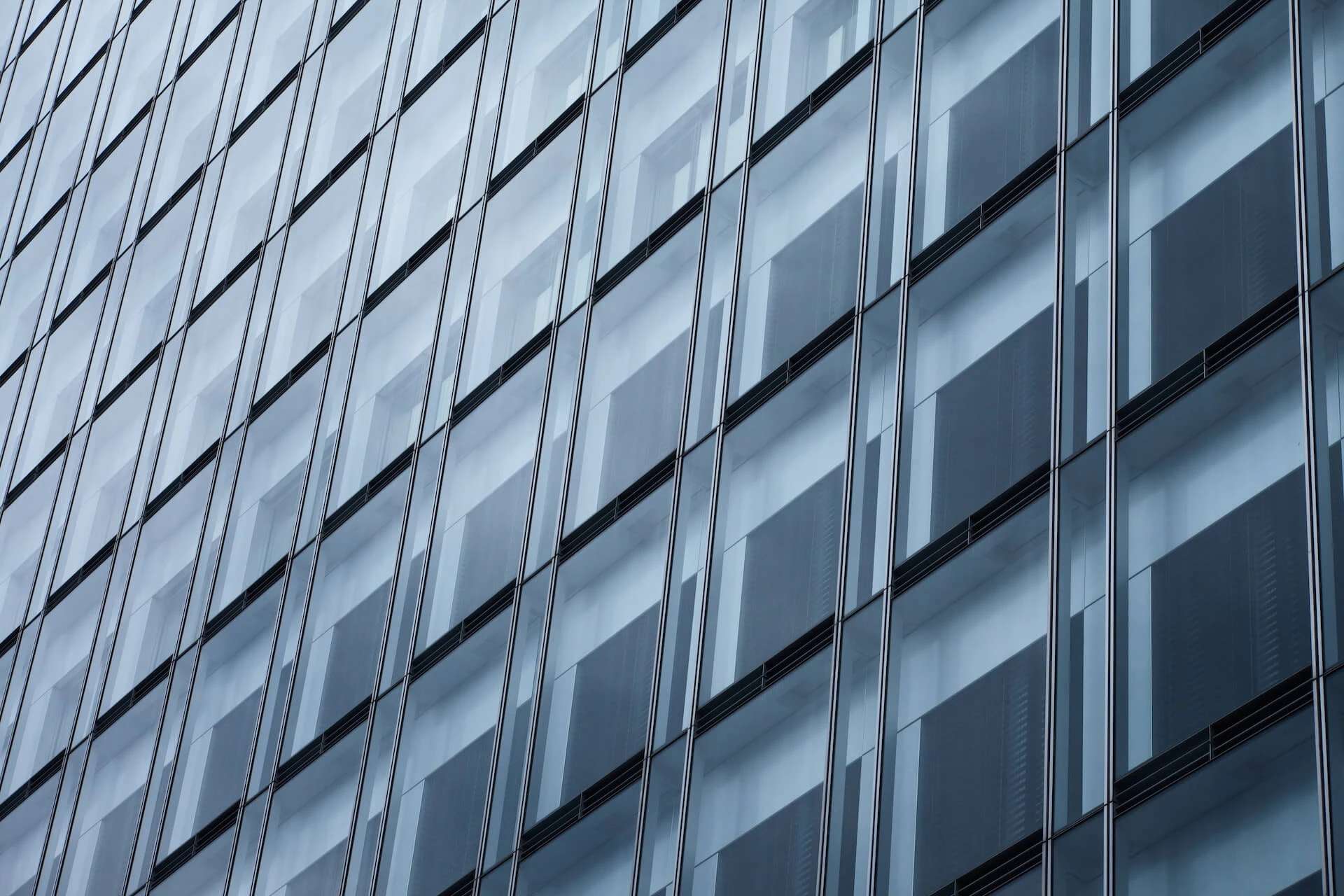
How to identify unreliable data?
Identifying unreliable data is crucial for drawing accurate conclusions and making informed decisions. If critical data reliability requirements are not met, you're working with bad data.
There are a variety of reasons why data quality issues arise in organizations. It can happen due to human errors, technical problems, external factors, and poor data management.
If you suspect data reliability issues, the problem is not yet identified, and you don't use automation that alerts you about these issues, paying attention to specific indicators in a dataset or file you're working with can point you in the right direction.
- Origin. Examine where the data comes from.
- Data collection method. Understand how the data was collected.
- Outliers. Look for values and other elements that fall outside the expected range.
- Inconsistencies. Look for conflicting or contradictory information.
- Missing values. High rates of missing data can be a sign of unreliability. Understand why data might be missing. Is it missing completely at random? Or is there a systematic reason?
- Historical data. If you have historical data, compare new data with it to detect any significant and unexplained changes.
- Duplicate entries. Duplicate data can skew results. Identify, investigate, and solve any repeated entries.
- Pattern recognition. For example, in surveys, if all answers follow the same pattern (like choosing the first option always), it might indicate unreliable responses.
Building products with reliable data
A company should be able to track and manage its data health. There isn't a single recipe for making your data reliable but a set of principles that help data-driven organizations continuously improve data reliability.
Data management policies that set clear standards and guidelines for collecting, processing, storing, and safeguarding data are key in building products with reliable data. Putting the work into these policies allows companies to ensure better data quality and security throughout the data lifecycle.
Like in any other industry these days, automation is one of the ways companies deal with data reliability issues. Automation contributes to better data reliability in various steps of data management, whether it's the actual processing of data you're sourcing or automated alerts that notify responsible teams about data-related issues.
If a company is sourcing data externally from a data provider, evaluating the reliability of the provider and its data is crucial. An experienced and reliable data provider will provide all necessary resources for testing the data before buying.
Paying attention to documentation is essential if you buy large-scale datasets, such as public web data on companies. Reliable datasets usually come with thorough documentation that describes how the data was collected, any transformations applied, known limitations, etc.
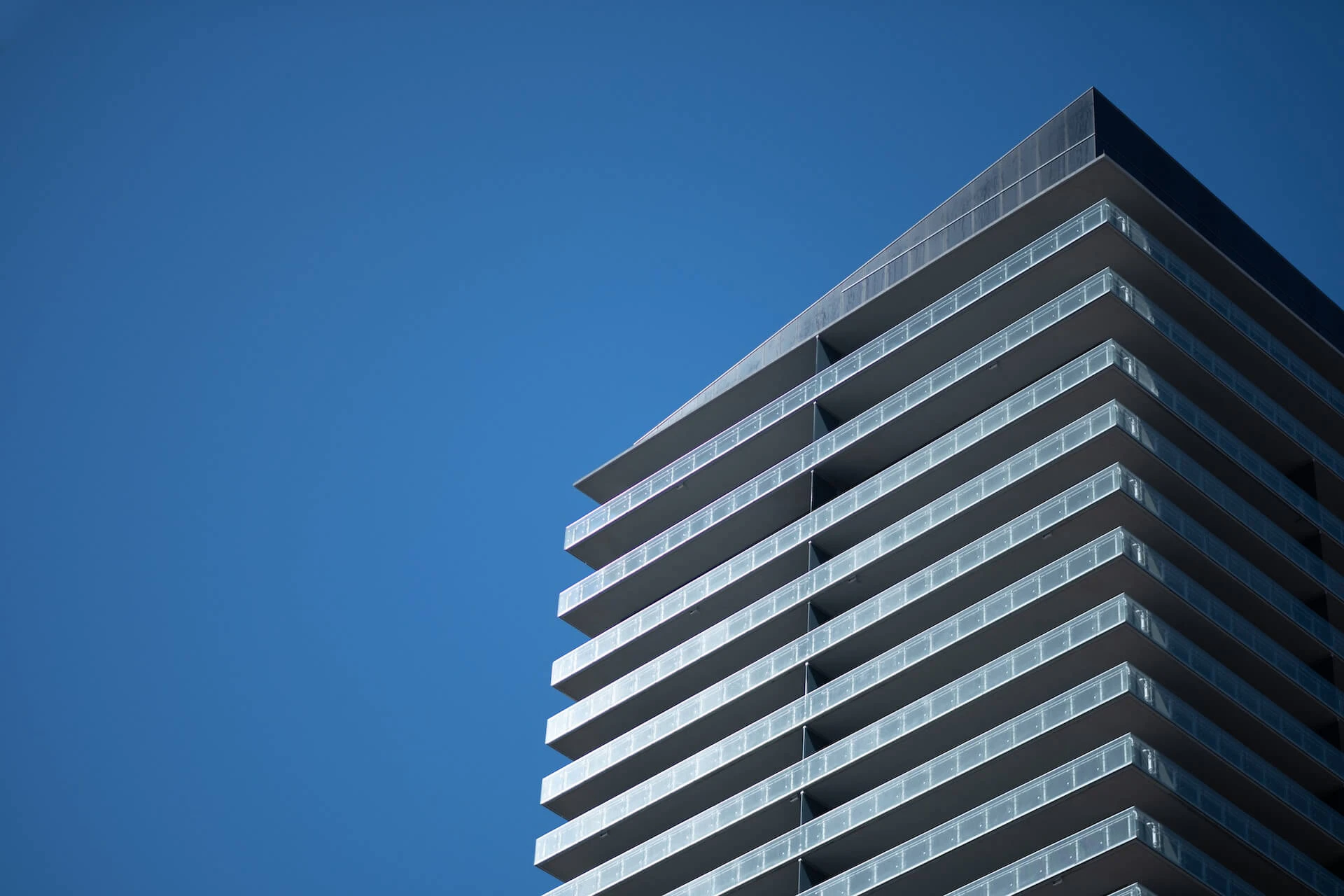
Why is reliable data worth the investment?
It's safe to say that reliable data is the only way to achieve substantial business results. Earlier in this article, we discussed how to ensure data reliability inside the organization, but many data-driven products rely on external data.
While an organization processes the data it buys based on its needs, the goal should be to source high-quality data that doesn't require vast resources because of poor quality. The data you're buying should be relevant and reliable. In our experience, 5 key questions help you select the best data provider before you buy.
Final thoughts
Lastly, as data is becoming more embedded in decision-making across the organization, data reliability should be at the top of the list of priorities.
More complexity introduces new challenges that need to be addressed. However, the ultimate goal is to use your data as effectively as possible, and naturally, data reliability is crucial for this. And if you don't have the right data yet, just let us know.